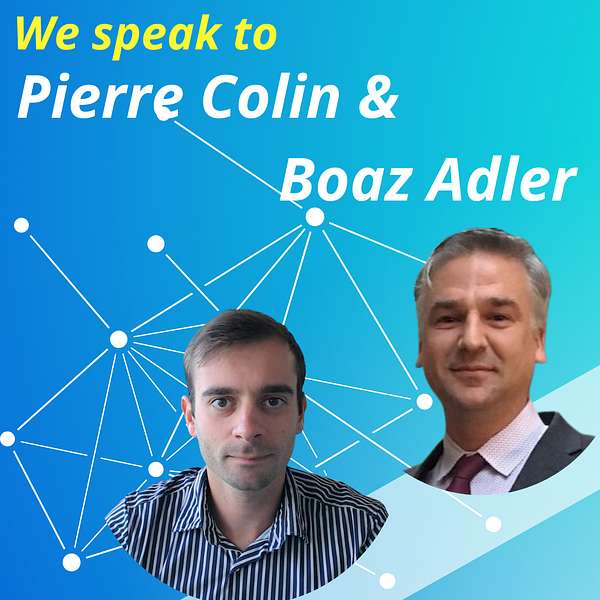
Transformation in Trials
A podcast about the transformations in clinical trial. As life science companies are pressured to deliver novel drugs faster, data, processes, applications, roles and change itself is changing. We speak to people in the industry that experience these transformations up close and make sense of how the pressure can become a catalyst for transformation.
Transformation in Trials
Innovating Clinical Trial Design with Pierre Colin and Boaz Adler
Unlock the secrets of clinical trial design with industry experts Pierre Colin and Boaz Adler as they join us for a deep dive into the early-stage planning that shapes the future of pharmaceuticals. They provide a look at the collaboration between clinicians, statisticians, and regulatory experts that's critical for determining the right patient populations, dosages, and study endpoints. With their guidance, we explore how statistical software and scenario planning play pivotal roles in estimating patient numbers, study durations, and costs, transforming clinical trial design into a fine art.
As we navigate through the evolution of statistical methods, our guests share their insights on the need for adaptability in clinical trial designs, especially in areas like oncology with unique endpoints such as overall survival. They spotlight the importance of cross-company collaborations and direct FDA discussions in driving innovations. The conversation also illuminates the ever-growing contributions of R-coding, a testament to the individuality of each study, allowing for customized and cutting-edge approaches to trial design.
To wrap up, we spotlight the dynamic landscape of drug development where biostatistics paves the way for groundbreaking designs. Our guests emphasize how feedback from scientific communities influences new methodologies, shifting the focus from traditional success measures to concepts like assurance and power in the promising zone. They also highlight the invaluable role of Bayesian designs, as exemplified by COVID-19 vaccine trials, in ethically accelerating drug development. As we close, remember that this field is ripe with diverse opportunities, and we invite you to reach out with your curiosities and follow-up questions.
Join us for this episode that's not just about the science of trials, but the transformation they undergo, ensuring the journey of drug development continues to revolutionize patient care.
Guests:
Pierre Colin: https://www.linkedin.com/in/pierre-colin-11139028/
Boaz Adler: https://www.linkedin.com/in/boazadler/
________
Reach out to Ivanna Rosendal
Join the conversation on our LinkedIn page
You're listening to Transformation in Trials. Welcome to Transformation in Trials. This is a podcast exploring all things transformational in clinical trials. Everything is off limits on the show and we will have guests from the whole spectrum of the clinical trials community and we're your hosts, Ivana and Sam. Welcome to another episode of Transformation in Trials. Today we're going to focus on the topic of how trial design is changing, and I have two guests in the studio with me I have Pierre and I have Boas. Hello, Pierre and Boas.
Speaker 2:Hello Ivana, Good to be here.
Speaker 1:And I'm very excited about these two guests. Pierre Collin is the associate director for biostatistics at Bristol-Mirage Scripps and Boas Adler is the global solutions engineer at Cytel. I'm really looking forward to this conversation. But to start us both off, I would be curious to hear from both of you how do you design a clinical trial? Which factors go into designing a clinical trial?
Speaker 3:So first, of course, we have to agree with the development team what is the objective of the study? There are multiple scientific questions we need to answer during drug development, like which dose should we use? How many times should we give the drug? Every week, every month? Should we combine the drug with another compound?
Speaker 3:Maybe a specific population would really benefit from the drug when similar patients that are slightly different may not benefit from the drug. So these are different questions, defining what is the objective of a new clinical study. And once we agree on what is the objective, we can discuss which kind of clinical data should we look at? We of course, always look at safety if any adverse events occur during the study, during treatment, but as a survival, early efficacy in oncology we may not wait for survival, so we always look first. If we were able to shrink the tumor and depending on which kind of data we plan to collect and what is the drug effect we expect to observe, we can derive how many patients we need to enroll in the clinical study. For how long should we monitor these patients to be able to collect the data we need and finally perform the final data analysis which will be used to discuss with health authorities if we can move to the next step of drug development or if the results are already good enough to support the drug submission for approval.
Speaker 2:The only thing I would add to that from my perspective in working with our clients is that it is a very complex system and putting together a clinical trial does take a lot of different perspectives and different aspects, as Pierre just highlighted, and so when we start working on a clinical trial we may have sort of a straw man idea of what that idealized trial might look like. But by the time we're actually launching that trial it may look completely different than where we started. So it's kind of interesting to see how those transformations happen as we're taking in information from those different perspectives.
Speaker 1:That makes sense.
Speaker 3:Yeah, typically, just to complete that, when you have a protocol for a new study, a statistician is able to rerun the sample size derivation to check how to perform statistical analysis within fewer hours, because there is just one protocol, one version of this clinical study. But when we start discussing about how should we do the next study and we have, as mentioned by Boas, a global idea of what we want to do, but we need to explore several strategies, several options, several scenarios and at the end we are exploring easily 30, 40 different designs and only then we can decide which one is the most convenient or which one leads to the faster study or matching being the most appropriate for our study objective. So very easy to say to explain it when we only have one version of the study design, but difficult to reach this final version because to do it we need to explore tens of different designs.
Speaker 1:And when would this exploration happen? How early in the process of planning of the trial?
Speaker 3:Very early. This is the first step and typically we don't know yet if we want to solve a dose selection, dose optimization problem or if we are looking to optimize the population, really targeting the smaller population benefitting the most from the drug, and we just try different strategies and at the end the development team or even the company leadership will approve one strategy or another one. But to be able to make that decision they need accurate assessment of what would be this new study how many patients, how long, how much. Of course we have to provide a cost assessment and basically we have to explore every design assuming this is a final one to be able to provide the full assessment to the leadership team, which will be able to make a decision and provide and share with us that decision so we can really complete the study protocol. So this step is very early because without clear endorsement of what is the strategy to apply, we don't know exactly how many patients we need, which kind of data we have to collect and at what time we have to schedule statistical analysis.
Speaker 1:I would be curious to learn more about how this is actually done. What kind of input would be collected to make this kind of analysis? Can you take us through the process of getting to those scenarios and then selecting one Sure?
Speaker 3:So you get a request from leadership. We want to target, we want to cure a disease. So there is a need, a clinical need as a clinic, and we believe that one of our compounds is would be appropriate and is promising. So we have first to discuss with clinicians typically biologists and physicians to understand what is this disease, what is specific to this indication, and then we discuss with regulatory experts. We can confirm which kind of data we need to collect, what kind, how much the drug must be effective to convince health authorities to approve a new drug. And once we have this information, we can start designing a simple study, usually just to give a high level assessment, and then we start looking for more complex strategy. Should we look for different levels? Should we plan for a subgroup analysis? And step by step we make the design more complex and we update every time how many patients we need, what would be the expected study duration, expected study cost.
Speaker 3:And to do that. We can, of course, derive, implement everything by ourselves, but this is when we usually rely on a statistical software, typically not the ones provided by Sector or other company. It's easy to implement multiple designs so we can compare quickly which one is the most appropriate. And it's at the beginning. It's really an informal discussion between clinicians, regulatory expert, statisticians, and then we can share, step by step, several recommendations with the development team, understanding what are the proven cons for each strategy, and we finally share our conclusions about each strategy with the leadership team, which will finally endorse the strategy we want to apply.
Speaker 2:I would just maybe add to that a little bit and thank you, pierre, for the shout out to our software. I do believe, and I agree with you, that our software really shines in those instances where you're looking to examine different methods and the ability to kind of layer all these methods side by side so that you can make some informed decisions, and those could be maybe a Bayesian design, if we're thinking about dose finding, for example, a dose escalation, or a more traditional design. So there's many options within the software to allow you to really have those early discussions and build that framework, if you will, for that final design. The only other thing I would mention that we spoke of earlier is looking at dozens potentially designs, potential designs, as we're working through this process but as you know, perhaps at Zytel we really advocate for even expanding that design space and looking at many more options and looking for those sensitivity analyses to the extent possible to find that final kind of typical design that we want to go with.
Speaker 1:Is there anything specific for oncology when designing studies that differ from other therapeutic areas? Is there any more rigour? Is there any more types of design that you need to consider?
Speaker 3:Yes, mainly quite different designs for early phase studies, because drugs in oncology are very toxic so we have to move very carefully when we explore multiple dose levels. We have to start very low, to enroll just a few patients and, depending on what we observe, we can discuss with physicians if it is safe and worthy to increase the dose level or not. So it's a really a step by step process and sometimes early phase study in oncology can be quite long, several years as long as a phase three study in another therapeutic area. So it's a very long, very cautious process because of the drug toxicity we observe in oncology. For the late phase it's mainly about the type of data we collect.
Speaker 3:In oncology the best clean-collent point which we use as a proof that a drug is effective and can really provide a benefit to the patients is overall survival. So the decent point is analyze a bit differently compared to what we use in diabetes, for example, or in our science. And the main issue is it is very long to monitor. We really have to monitor patients for five, six, sometimes up to 10 years and therefore phase three studies in oncology are very long and this is something influencing the statistical design we have to consider because basically no one can afford to wait 10 years before the first statistical analysis. No patients, no physician, no sponsor can wait so long. So we have to plan for successive analysis over time, typically one analysis every two years to be able to check if we already have vaporizing results and if these results are good enough to start discussing with health authorities to be able to fasten the submission process and to be able to to get the drug approved as soon as possible.
Speaker 1:What did you have a comment?
Speaker 2:Yeah, I would just add to that that, in terms of those interim analyses, I think the timing of those is very important and that, again, is something that you can do very easily with existing software. But more than that, and just to expound a little bit, I think another area that is of interest, maybe specifically to oncology, is looking at subgroups and looking at maybe we're targeting specific genes, especially in more crowded areas, such as non-small cell lung cancer or, if you're thinking of breast cancer, we're looking at smaller and smaller populations, so we're no longer I mean some, I think some drugs and some designs look at a broader population, but we're really looking at more and more targeted therapies, and that's very much an area of interest and there too, finding those patients and being able to design a trial that can accommodate that smaller, perhaps, subgroup is very much of interest.
Speaker 1:Are there any typical trial designs that emerge that you can reuse or time, or would it be a unique situation every time?
Speaker 3:Almost unique situation. We use, of course, the same theory, the same statistical methods. So typically, when planning successive analyses we usually rely on what we call group sequential design. But situation is always a bit different. Clinical assumptions are different, timing of analysis maybe always a bit different, because we never look at only one clinical endpoint, we always look at four, five, ten different endpoints, and some endpoints are collected and available much faster.
Speaker 3:And we would like to match an interim analysis for overall survival with the final analysis of an early endpoint, typically response rate in oncology, to determine how many patients successfully shrink the tumor size. And depending on the schedule of the tumor scan, depending on the type of cancer, these analyses may happen at a different time. And then we have to adapt the timing of interim analysis for survival same if we want to match progression, free survival and overall survival analysis. So we are always relying on similar methods, but we are always updating clinical assumptions and statistical assumptions depending on each indication, each study, so we cannot just copy based when it was done for another study. There is always something different and the difference is significant enough to make us running against the whole process and is there any sharing of ideas or cross-pollination between different companies working within the same disease areas?
Speaker 3:Sure, we have cross-company working groups. Of course, we share feedback during scientific conferences, either medical conferences or statistical conferences. There is also an interesting program from FDA to submit for very innovative design and not being sure if this innovative design is acceptable by health authorities, and in that case FDA is offering additional meetings. These meetings are actually public and open to anyone and it's a way to discuss a new design, because we don't have yet any actual example, a true study, being implemented with this design. So it's an opportunity to discuss directly with health authorities to determine if this new design is good enough and acceptable to answer a new scientific question. And for the sponsor submitting this new design, it's, of course, an opportunity to collect a lot of feedback from health authorities and for anyone else, it's the opportunity to to collect some ideas for future study.
Speaker 2:We definitely see those seminars and and conferences as a very fertile ground for collaboration and interest in conversation between academia, the industry, software as well as pharma. So I see it as a very collaborative process for sure, certainly in method development and method acceptability.
Speaker 1:And how do those methods evolve over time? Does it come from academia? Does it come from the industry? Does it come from vendors? How does the evolution happen?
Speaker 3:Well, first a bit of everyone. It's practically we have four different participants universities providing publications about innovative methods. We have software companies providing a tool very useful to implement these innovative designs, because otherwise we would have to implement the old method by ourselves, so programming the exact mathematical formulas, because it's very time-consuming to validate these kind of programs. Of course, pharmaceutical companies proposing a new study. We can always propose a new design when submitting a proposal for a new study. And finally, health authorities publish quite often guidance about new methods or at least new issues raised over the last decade in drug development, and it's always difficult to understand which one. Which participant has to move first.
Speaker 3:Of course, usually university would publish about a new method, but then it's difficult to implement these innovative methods without the support of software company. But software company are not that difficult for them to to work on these innovative methods without knowing if they will have customers to pay for it. Sometimes companies, pharmaceutical companies, wait for the new guidance for health authorities before really working on a new study design. So, depending on this situation, maybe software company will provide a new tool and it will become very popular and we will start using a new method that way, or sometimes health authorities will not make a new method mandatory, but we'll definitely advise to use it and sometimes pharmaceutical company will submit a new study with a new design and this would become a very interesting example for everyone else. But of course it's difficult to implement a new study, a new design, so other sponsors may prefer to wait for software company to provide a validated tool before really using this new design.
Speaker 2:From a software perspective I would mention we have seen the proliferation of the use and kind of normalization of the use of group sequential design, certainly in some cases promising zone methodology, as it appears within east and solar, some of Seytel's products.
Speaker 2:But, as Pierre mentioned earlier, I think each study is very unique and tailored to the patient population, the drug that is being developed and so on, and so there's always this struggle to fit the different pieces and the uniqueness of each one of these studies within the existing methodology, within the software. One of the kind of exciting things that are coming this year we started this last year at Seytel, but coming even more this year would be the introduction of our coding into the software directly, so that you can use some of the hard-coded and validated sort of designs and methods that already exist within the software but be able to then tweak that design with the specific methodologies or the uniqueness of that study. So that is one of the ways in which software companies, certainly Seytel, are looking to address this need within the industry and maybe break that cycle of the chicken and the egg. Right, who's the first mover in terms of that method and what is going to be acceptable?
Speaker 1:And if the new methodologies have not been implemented in the software yet and from a suitable company still does want to use the new method, how would they go about doing that?
Speaker 2:Currently I think the kind of off the shelf solution would be to use R-coding, and certainly Pierre would know a lot more about this and hopefully can comment. But, as I mentioned, with the opening of this collaboration, of pulling in R-coding into the traditional software, I do believe that in the coming year or so we would be able to accommodate more and more of those methods. And these methods could be as specific as maybe driving for a specific metric for assurance or probability of success. It could be addressing how we account certain patient events, things like that. So a more minute methodology to make those data decisions within the study. But, pierre, you probably have more background there. Yeah, definitely.
Speaker 3:When a method is not available in commercial software, we have to implement it internally, so a kind of homemade solution.
Speaker 3:It's time-consuming, it requires a long validating process to make sure there is no mistake, and we can use it to submit a new protocol and then to support statistical analysis.
Speaker 3:Of course, when we do it it has to be shared with health authorities so they can rerun everything and check that the program we used is correct. Hopefully this scenario is not yet a modatory, but it's becoming a common practice in universities, when publishing an innovative design, to publish our package as well at the same time, so that we already have a tool to implement this new method. It's not yet as convenient as commercial software, as is Solara or Encrypt, but it's already good enough to explore a new method and at least to document which tool we use. And since one very critical requirement is to be able to rerun exactly the same way a statistical analysis for quite a long time, usually 10 to 15 years so we have to document very accurately which software we use. If we used additional packages, we have to list all of it to make sure that anyone else needs statistician from health authorities or maybe another statistician from the same company taking over for the program would be able to rerun everything smoothly and without support from the previous statisticians.
Speaker 1:Could I ask for an example of a new methodology that is relatively recent and how that may have changed? Trial design.
Speaker 3:There's one not yet applied commonly, but it's similar to group-selection design but on top of that we can select a subgroup of interest. So let's say we start with several subgroups and at interim analysis we do not only check if the drug is promising or not, we also check which subgroup benefits the most from the drug. And if one subgroup doesn't benefit from the drug we can stop on welding patients from the subgroup claiming. It's just a waste of time for everyone, both patients and sponsor. But we can carry on and enroll more patients from the remaining subgroups which seem to benefit from the drug. This is very convenient because then we can apply for an overall sample size. We don't know yet how many patients we will enroll from each subgroup, but we know that at the time of end of study we will be able to make a decision and to support drug approval if the results are promising enough.
Speaker 3:And it's very flexible, very convenient compared to planning two parallel or three parallel studies, one for each subgroup, and analyzing each study independently from each other. It's very time consuming, time consuming. It's much more expensive compared to this new design. We call it group enrichment, sequential enrichment design, because we are able to perform sequential analysis and to select the subgroup of interest within a single study. So it's very, very convenient. Difficult to implement, especially when analyzing survival data, but when we have an early endpoint we can use to check if the drug is effective. This new design could be very interesting because we can use the early endpoint to select the most promising population and still enroll new patients from this subgroup until we can finally analyze the long term data, which usually are survival data.
Speaker 2:Subgroup. Enrichment, as you're describing here, pierre, is actually a great example of one of those methods that we've been hearing more and more about within SITEL from our clients that there's more and more interest for all of the reasons that you've just mentioned, and it's a good example of a way that the industry then affects the software, because we have implemented the plans to put enrichment in place as part of the methodologies that are being launched in 2024. So that's one of the ways in which the four areas because, if you will, in study, development, method development interact together. So that's a good example there.
Speaker 1:And what about the regulatory side of things? Are the regulatory agencies making it easier to implement new designs? Are they putting barriers in place? What kind of role are they playing?
Speaker 3:Well, of course they require proof that an innovative design would achieve the classic requirements for a clinical study Low probability of success when the drug does not work and high probability of success when the drug is effective, to be able to select properly the dose or to select properly the subgroup of interest.
Speaker 3:And the more complex the design is, the more difficult it is to provide mathematical proof for these requirements. So now health authorities are willing to accept a simulation study instead of mathematical proof. So it's providing mathematical formula proving that typically what we call type one error probability, to observe a study success when the drug doesn't work, we can now do it by simulation. We would simulate like 1 million clinical studies, assuming that the drug doesn't work, and we will check out of 1 million studies how many times we observe a success. And for complex designs it's sometimes the only way to estimate what is a type one error probability. And it's now. If we have a large number of simulations. Of course it's now good enough to convince health authorities to apply these new adaptive designs. Otherwise it's difficult to provide a mathematical proof and therefore difficult to convince health authorities to accept the new design.
Speaker 2:I would add that, as you know, monte Carlo simulation is at the heart of all of our software here at Cytel and certainly we know that the regulator appreciates when maybe the part of the study package includes those East files or easily readable files and shareable files where they can see those simulation results. So again, different ways of using the software to inform either the regulator or the manufacturer submitting that study design.
Speaker 1:When you mentioned this vast amount of simulations that you would actually look at and look for success rates, it sounds like it's rather, that it would consume a lot of both computing power and also be heavy on the infrastructure side. What does it actually require to run this amount of simulations?
Speaker 2:Maybe I can take the first practice here Definitely takes a lot of simulation power. Oftentimes and traditionally we've seen our pharma partners using their own internal resources, their own servers, to run these very large simulation runs and those could become bursam and take some time, depending on how much of that computational power is available within a particular company. What we're seeing more recently is a move to cloud resources rather than on-prem resources for simulation and certainly with the advent of Solara about three or four years ago now, we are seeing that implemented within the software directly. So part of the solution is not just the methods and those kind of workflows that are built into the software, but also the simulation power. The computational power is available within the software and with it being in the cloud we're also seeing it being much more efficient and a much quicker turnaround time on those simulation results. Curious, pierre, for your perspective as well.
Speaker 3:There is a saying in Informatics that our current smartphones are actually more powerful than the computers which sent spaces to the moon decades ago. It's actually true, but the more computing resources we have, the more complex methods we can explore, and it's a loop the more we have, the more complex we can explore again and again, and it's always time-consuming to run simulations with complex adaptive designs. And there is no hand. Even if Informatics improved again and again, we will always imagine more and more complex designs for studies.
Speaker 3:And this is when clusters or cloud-based clusters are very convenient, because then we can access a kind of cluster equivalent to tens, sometimes hundreds of computers, and we can run simulations in parallel.
Speaker 3:And instead of asking one computer to run one million simulations we can ask hundreds of computers to run much less simulation each, and that way we can really shorten the time we need to wait for the results.
Speaker 3:And it's very convenient in drug development, because when leadership is asking for a new study we cannot afford to wait weeks just to go to house and simulations went and if the results are good looking or not. So it's very convenient to have either efficient commercial software or to access cloud-based clusters so we can really run simulations faster. Typically we can afford to wait several days at most, but anything longer than several days we would give up. Because if we have to wait like one week just to explore one design, then we go back to the development team to discuss this design and a new idea we will raise and we will have to explore a new version of the same design and then a new one again and again, and at the end we will need months just to agree on what design will be appropriate for the new study and it's just too long. We cannot afford that. So parallel computing it's for plastic design is fine. We may not need it, but if we have to explore complex design by simulation, parallel computing is a must.
Speaker 2:And just for a scale, if you will, if in the past, if using internal resources would have taken maybe a few hours a day or maybe even several days to run a complex design via simulation Within Solara. Today, for example, we're looking at 200,000 cores that could be fired up at the same time if need be. So it's flexible, where you're not using all of those computational resources every time. You're just opening up those resources as needed and typically for a study design. That all of the study designs that I've worked on were, and most of them were less than 30 minutes to get that turnaround information. In some extreme cases, where it is a much more complex design, maybe up to 45 minutes to an hour, but the turnaround times using these more flexible solutions are much, much quicker than before and, to Pierre's point, being able to turn around iterations over and over again is really what the process looks like. So having that in your back pocket and having the ability to iterate quickly is a must.
Speaker 3:We can even run designs we don't need, just in case Typically the clinical team is not sure about one assumption when we want to analyze survival data.
Speaker 3:We are not sure about the median survival time and we have many assumptions.
Speaker 3:Sometimes it depends on which population we will really target and roll in the new study.
Speaker 3:So we can duplicate and try every combination and typically one side just derive like 40,000 different designs by combining multiple values for multiple assumptions, because we were not sure which value we would finally consider. And that way, thanks to some tools similar to the case so you can run thousands of designs within one day and then you have everything you need and even if the team is providing you with an updated clinical assumption, you already have the design you need because you already explore multiple values for different assumptions and you don't have to rerun everything and you can already support the discussion with the development team during the same meeting, instead of going back to your computer running all calculations or simulations again and scheduling a new meeting the next day or the next week, so being able to run a lot of simulations ahead of schedule. Thanks to these cloud-based or parallel processing software, it's very convenient to save time and to anticipate a different scenario or a different design. Otherwise, for the sake of time, we would have to wait feedback from the team before exploring a new version of the design.
Speaker 1:It sounds like more computing power here can actually really accelerate our drug development timelines.
Speaker 3:Definitely. And even drug development is long. You really let's use a very high level estimate let's say a decade to fully develop the drug. But at the end, if we save just several months, it's already significant for a sponsor to invest in a new tool, a new equipment, a new method, because, at the end, being able to reach markets several months ahead of schedule it's really significant for the company. And it's good enough to support software provided by companies like Sightail, to support innovative design or to invest in computer clusters.
Speaker 2:And we have seen with those customers that we've implemented Solara as a solution with, specifically, the really shortened turnaround time and the ability to reach that final decision and that statistical protocol much quicker than they were able to do in the past. And we are shortening by months, sometimes weeks, certainly months, the turnaround time for those final designs. So, to your point, sometimes get reaching market just a few months sooner than you would have been traditionally can make a big difference both for patients and from market perspective.
Speaker 1:We've talked a lot about developing new methodologies. Are there any methodologies that tend to fall out of favor and stop being used?
Speaker 3:In Okraji. I have one main example in early phase, what we call dose escalations. Today we don't know yet which dose level we should use, so we start very low and step by step we explore higher dose levels. There was one design called 3 plus 3, just because we plan to include patients by small cohorts of three patients. It's very simple to implement. But over the last 20 years we observed quite a large number of publications showing that this design is definitely not the best, if not the worst, and we now most of sponsors, move to what we call model based designs, such as continuous reassessment method or escalation, with over toxicity or over dose control, using all available data to predict what would be the safety we could observe if we decide to increase the dose. These designs are more effective and now it's definitely supported by health authorities advice to switch from 3 plus 3 design to model based design. Of course it's a very long process. The first publication for model based design was published in 1990, so more than 30 years ago. But we cannot switch right after publication.
Speaker 3:We first asked to explore the method, to try to have a kind of virtual study to understand how the CDE would behave, assuming we would implement a new method, even if in practice we do not, and step by step, we collect feedback from everyone, from different scientists, from universities, but also from private companies. At some point, health authorities will update a guidance document to provide their opinion about these new designs. Sometimes the health authorities are the one triggering the process by claiming look over the past decades. We have this issue raising again and again. So anyone having a proposal, please jump in and explain what would be a new method to deal with this issue, because so far we don't have an appropriate solution and design. It can be submitted by other universities or by private companies. It's like a call for abstract. If you have any idea, please jump in and let's go and we would start a walking route to share experience, to work together to determine if this idea could be converted into an actual innovative design.
Speaker 2:And I would say that dose escalation is actually, or the evolution in dose escalation, is really a good example of how both academia and the regulator helped shape what the software is then able to offer and being able to maybe benchmark on a traditional 3 plus 3, as Pierre described it, but also then comparing it to several other frequentist and Bayesian methods and seeing how all of these align next to each other for that particular study design is a very powerful tool that we see our our clients use, both with East and East base.
Speaker 2:Another aspect that I, if I could add, in terms of maybe things falling out of favor or being less popular, I think in the past there was a very heavy focus on study power as a predictor of study success, and we're seeing somewhat of an evolution in terms of the understanding of what study success might be and looking at other measures for how we define that probability of success. Perhaps we're looking at assurance, which is again bringing in more novel Bayesian methodologies, more informed strategies, or looking at power in the promising zone, other and other yardsticks for success, if you will. So that is one area that we're seeing a big change in how our clients use the software.
Speaker 1:And are there any barriers standing in the way of potentially taking even more innovative trial designs into use?
Speaker 3:Yes, of course there was a barrier for quite many years regarding Bayesian statistics, since for Bayesian statistics it was difficult to provide mathematical proof of design properties. But since now they're willing to accept a simulation study as proof, we can move with Bayesian statistics and that until now we were assuming. Okay, we can use Bayesian design, but only for published phase studies. And I recall one colleague, from Novartis I guess, claiming that we would have to wait for statisticians working at health authorities to get retired before so that the new one may have a different opinion. But actually we didn't have to wait that long because if we look at the phase three studies for the new vaccines against COVID-19, so during the pandemic, some of them were implemented using a Bayesian design.
Speaker 3:I think that the Pfizer phase three study was implementing the group sequential design but using Bayesian statistics instead of the frequentist approach. So it's nice to see that we can now explore these new methods. To be fair, most of the time it's exactly the same. We can have exactly the same decision rules, the same statistical analysis, hopefully, because there is no reason to have something different just because one statistician decided to use one specific method instead of the classic one. But sometimes Bayesian design are very useful to combine different sources of information, to combine data we collect during the clinical study with data already collected during a previous study or during a real life.
Speaker 3:It's especially convenient when it's difficult to enroll patients, when the disease is where we can replace some of the patients being treated with a placebo by patients we already treated during a previous study with the same sort of care and because we would assume we would observe the same outcome on average and that way we can make the study faster and a bit cheaper sometimes, because we benefit from previous data and we don't have to start everything from scratch every time we want to study a new compound. And this new approach is now supported and advised by her societies, so it's another innovative approach we are looking at more and more.
Speaker 1:That is very exciting. I love that statistics can actually help us both avoid additional unnecessary human trials, but also benefit from data that we have already. That is so exciting.
Speaker 3:It's a regular issue raised by ethics committees, of course.
Speaker 3:Why should we treat so many patients with a standard of care when we already know that standard of care is not that effective?
Speaker 3:It's a waste of time, it's a waste of resources for the sponsor and it is, of course, a waste of clinical opportunities for the patients.
Speaker 3:So by benefiting from data already collected during a previous study, that way we can make sure that we treat as many patients as possible with the most innovative and more chromizing drug.
Speaker 3:But when we have to do it from scratch and we cannot benefit from previous experience, statistics tell us we have to keep a balanced round-upization so to include as many patients in both treatment arms and, thanks to historical data, we can decrease number of patients in control arm and offering more opportunities to patients who usually want to benefit from the innovative drug. That's the main advantage of taking part in clinical studies to access an innovative drug which may be approved only in four or five years, even later. So, of course, without guarantee that the drug is more effective than standard of care. But if it is, you save so much time instead of waiting for the final approval, and for many patients it's the difference between staying alive and death. So it's definitely something that ethics committees push for to be able to offer this opportunity to benefit from the new drug instead of enrolling too many patients in standard of care.
Speaker 2:To Pierre's point, I think biostatistics is one of those areas where innovation for the sake of innovation may be academically interesting but very specific to the particular situation. And so to the extent that thinking of limitations, to the extent that innovation can lead to either better data or more prolific data collection and assessment, I think that's where that innovation really applies and is useful.
Speaker 1:I would be curious to ask both of you if there was some inciting incident for both of you when you realized, hmm, this is a very interesting field. This is the field that I want to work in.
Speaker 3:So to work in medical research.
Speaker 3:actually, to be honest, I wasn't aware I could be a statistician in medical research until I was very, quite old 22, so almost completing my education program and I just realized during the last year we usually get specialized in one specific field and when I started to study statistics, I thought I would work in finance or insurance company and I realized that actually we can do a lot of science and mathematics and statistics in medical research and this is something that we are not aware when we start our education program and it's a pity because it's definitely very interesting, but it's again.
Speaker 3:It's never the same situation. So even if you keep working on the same kind of studies, there is always something new for the next program, something slightly different. You have a new requirement from the team and you have to think again about how to deal with this new question, new requirement, and there is always something new. So you can basically you can work 10 years on the same kind of clinical studies and still learning something new every time, and after that, if you feel you learn all of it or almost, you can switch to a different kind of, a different phase in drug development, starting with early phase, and after five to 10 years you decide to work on late phase, or you can switch to a different therapeutic area, working on a different disease. So it's definitely not boring and we can work full career and still having something new to learn.
Speaker 2:Similar to Pierre, I think the kind of drug development process is very hidden from when you're just starting off and thinking of careers and it's something many people maybe wander into or fall into over time and perhaps telling Lee a few years ago I went to Career Day and my son's had a school and all of the children gravitated towards the mom who's the firefighter or who's the doctor, but nobody wanted to hear about drug development for me. So perhaps you know we can do more in terms of STEM education and encouraging and making this more publicly available so that we can understand that the many different areas within drug development that you can touch and we certainly spoke about the kind of multi discipline area approach that is required to design a trial and then bring that drug to market. And, similar to Pierre, it's something that I've kind of fell into over time. Certainly I'm glad to have found it because, also, I see the kind of ability to both learn about innovation, help promote that innovation and learn something new every day on the job.
Speaker 3:Typically to work on a clinical study. We have 10 to 15 different jobs and suddenly for drug development, then you can discuss about drug production legal department. So we are typically at BMS. We have some kind of open doors event bring your kids at the office or inviting first year students from one university to come and visit our facility and to discover how many different jobs we have, because the pharmaceutical industry is not just about medicine and chemistry. We have 10s of different jobs, but most of people and most of children are students, are not aware of this job diversity and we have to work on this kind of events to bring them into your face and introduce the different types of jobs they would like to apply for five or 10 years later.
Speaker 1:Well, I have a few. The same way, I also fell into this industry and I love it, and we'll shout from the rooftops how great it is to actually work within our space and how many different opportunities there. As we start rounding off, we always ask our guests the same question in the end, and I'll ask it to both of you If I gave you the transformation trials magic wand that has the ability to change one thing in our industry, what would you wish for? And let's start with you, Pierre.
Speaker 3:Sure, two things, one very personal. I performed my PhD in statistics on model based design. For those escalations to the you know courage, so typically the three plus three versus model based design. But I was trying to improve the model based design already published and to convince people to use extended version instead of the original version.
Speaker 3:And I realized that most of people were still thinking about switching from the three plus three to the model, the original version of model based design. So, but I was already 24, 25 years old and realizing how much time. Basically, I was born almost at the same time as a model based design, in 1919. And 25 years later people were still thinking about switching from one method to the next one and it was very frustrating to see how long it is and how we can we can make it faster to implement from one tenured professor publishing a new method until we finally implemented into a true clinical study to support the development. And to do that we need a much more efficient collaboration between pharmaceutical companies and universities.
Speaker 3:The second point it's a very innovative design, difficult to implement and something we are not yet clear about exact properties.
Speaker 3:But it's called multi arm, multi stage design, starting with multiple drugs which are all of them are promising to cure the same Disease, is the same kind of patients, but we don't know yet which one is the best. And if we do it independently, we would have one study to compare one drug versus standard of care, then another study to compare another drug versus the same standard of care. Again and again we would waste time and resources by enrolling multiple times the very same control group with the same standard of care. Drug and multi arm, multi stage design would be useful to save time by putting typically gathering two or three different sponsors and let's say, okay, we are targeting the same population. We know that we will have to compare our innovative compound versus the same standard of care. Let's have a joint study and made the best drug wins, but it's difficult to implement in practice and difficult to to explore to make sure we carefully understand the properties of these complex designs.
Speaker 2:If I had one wish, I would. We've talked quite a bit about collaboration within the industry and the four kind of main pillars of innovation within clinical trial design. Anything that we could do to increase that collaboration, that conversation, the communication between those four main pillars, I think would be of benefit to all of us and I welcome any suggestions out there. And since Pierre had two wishes, I will also wish for world peace.
Speaker 1:Nice. Well, if, if our listeners have any follow up questions to any one of you, where can they find you and ask more questions? Maybe, boys, you go for this first time.
Speaker 2:Sure thing, my email address I think might be included in the body of the text for this podcast can also find me on LinkedIn. I'll be all over the place in conferences, webinars, seminars this year, so I'm sure our paths will cross, if that is your wish.
Speaker 3:Some of us was email address and making us are the best way to contact me. Unfortunately, for those in US who may listen to us like located in Europe, so you may not see me in a conference in US usual practices to travel in within your continent and avoiding very long trip across the world, especially for big pharma companies such as Bristol Myosquid, we have facilities in US, in Europe, in Asia as well, so as a company we attend a lot of conferences but we send in colleagues in local conferences, so I may not be able to join the conference in US, but still you can reach me by email or with Tim.
Speaker 1:Awesome. Well, thank you both for coming on the show. I really love this conversation.
Speaker 2:Thank you, ivana Pierre. It was so much fun to get to know you a little better. We'll keep in touch.
Speaker 3:Likewise Thanks both of you.
Speaker 1:You're listening to Transformation in Trials. If you have a suggestion for a guest for our show, reach out to Sam Parnell or Ivana Rosendale on LinkedIn. You can find more episodes on Apple Podcasts, Spotify, Google Podcasts or in any other player. Remember to subscribe and get the episodes hot off the editor.