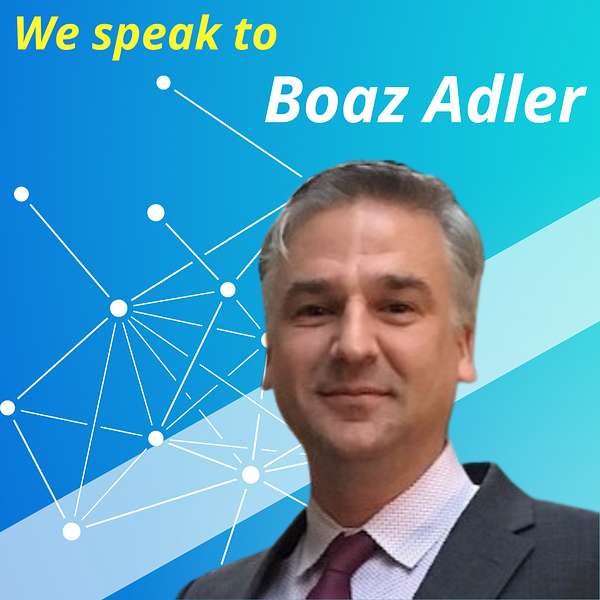
Transformation in Trials
A podcast about the transformations in clinical trial. As life science companies are pressured to deliver novel drugs faster, data, processes, applications, roles and change itself is changing. We speak to people in the industry that experience these transformations up close and make sense of how the pressure can become a catalyst for transformation.
Transformation in Trials
“Adaptive Clinical Trial Design: Large-Scale Study Simulation to Design for Results with Boaz Adler
This week we speak to Boaz Adler from Cytel's Software Division. This episode promises to illuminate the intricacies of trial design through the lens of Monte Carlo simulation, revealing how this potent approach crafts trials resilient to a myriad of scenarios. Boaz delves into the pivotal roles of interim monitoring and strategic market positioning post-trial, offering a masterclass in the art of clinical trial conception that withstands the test of uncertainty.
As we navigate the vast landscape of clinical trial simulation, the topic of scale takes center stage. The conversation orbits around pioneering software platforms like Solara and East, which are reshaping trial design by integrating a kaleidoscope of variables and uncertainties. This episode pulls back the curtain on the potential of cloud computing to revolutionize simulations, providing a glimpse into the future where clinical trials are executed with unparalleled speed and precision. With Boaz' expertise, we ponder the industry's readiness to trust probabilistic outcomes and how regulatory bodies are warming up to the simulation-based approaches that these software platforms enable.
Finally, we bridge the gap between biostatistics and market access, highlighting the increasing influence of health outcomes research on clinical trial design. The discourse delves into the balancing act of incorporating quality of life measures for regulatory and reimbursement decisions, the finesse required to blend standard software with bespoke coding, and the imperative of enhanced inter-departmental synergy. My own path to life sciences underscores the episode's reflective tone, leading to a broader examination of Cytel's extensive contributions to the field, from their roots in Monte Carlo simulation software to a comprehensive suite of services that spans the full spectrum of statistical programming and evidence-based research. Join us for this enlightening episode to gain an insider's perspective on the dynamic confluence of biostatistics and pharmaceutical innovation.
Guest:
Boaz Adler
________
Reach out to Ivanna Rosendal
Join the conversation on our LinkedIn page
You're listening to Transformation in Trials. Welcome to Transformation in Trials. This is a podcast exploring all things transformational in clinical trials. Everything is off limits on the show and we will have guests from the whole spectrum of the clinical trials community and we're your hosts, ivana and Sam. Welcome to another episode of Transformation in Trials. Today in the studio with me, I have Boas Adler Hi Boas.
Speaker 2:Hi Ivana, it's such a pleasure to be here today. Thank you for inviting me.
Speaker 1:I am so excited about this episode. Now, Boas is a Solutions Engineer at Zatel's Software Division and we'll talk more about Zatel and their software later in the episode. But today we're going to focus on a super interesting topic, which is simulating studies at large scale to design for results. And before we really get into it, Boas, could you tell us more? What is trial simulation?
Speaker 2:Absolutely, and I understand that maybe many of your listeners are not necessarily biostatisticians or in the business of designing clinical trials.
Speaker 2:So it's, I think, a really good place to start.
Speaker 2:And what we mean by trial simulation is, at least, on Zatel's side, we utilize a technique called Monte Carlo simulation, which is a risk analysis technique that is used to deal with very complex, uncertain models, and so, instead of representing specific values within that model, it takes a range of values, or what we call probability distributions, to represent different possible inputs into that model.
Speaker 2:In this case, what we're talking about is the model here is really that clinical trial design. So you can imagine, when designing a specific clinical trial, there would be many different inputs that go into that model and many possible outcomes of that model, and so using Monte Carlo simulation is really very well suited for this type of analysis. And then the last thing I would mention is that we use computer power to calculate all of these different ranges, all of these different combinations of ranges, to get at what is the most likely outcome, in this case for a clinical trial. So that, in a nutshell, is Monte Carlo simulation. And Monte Carlo simulation, it's important to add, is sort of the bread and butter of the industry when it comes to clinical trial design, and it is the type of simulation that is embedded in all of our software solutions here at Seidel.
Speaker 1:Hmm, that's awesome. Maybe a stupid question, but I'll ask it anyhow. What if you do not use simulation for planning your trial? What does that look like and where does that leave you?
Speaker 2:Yeah, and you know, early on maybe a few, I don't know decades ago it was completely acceptable to just use computation rather than simulation to design particular clinical trials. So when you think of your traditional trial where you enroll patient into the study, you follow them for a certain period, you collect some data and then you complete the study and read that data kind of unblind, that data computation works just fine. What happens is as you make this model, this particular trial, more complex, using more advanced methods for adaptation of the study say adding an interim analysis to your study or considering re-estimating the sample size of your study mid-trial that's when things become a lot more complicated and at that stage computation is no longer a valid way to assess whether a trial will be successful or not. And that's when those simulations come in handy to help kind of predict the outcomes of your study, including all of those adaptations.
Speaker 1:So this would be a way to make sure that we can make sound decisions before actually starting the trial, or do we also use it as we go?
Speaker 2:Absolutely so. It is a way for us to make sound decisions about the trial design itself. It also allows us to design trials that are more robust to that ultimate range of possibilities of, say, your treatment effect or your enrollment rate. We know that there's a lot of uncertainty about what that true underlying treatment effect for a particular product would be, and so being able to design a trial that would perform well under more positive or more negative scenarios of that treatment effect is really what we're aiming for. In terms of a during trial execution, we can use some of the software to do what we call interim monitoring, so taking in the information that was collected so far and using that to help predict the future of that trial as it is ongoing. But I would say the majority of the work, at least, that I do with clients is Monte Carlo simulation for trial design. So still in the design stage, Well, that makes sense.
Speaker 1:And what do we model when we model a trial? Is it specific endpoints, parameters?
Speaker 2:Absolutely so the type of inputs that we would consider is yes, what is the? What is that scientific question that we're looking to resolve? What are those outcomes that are important to us for a particular product or for a particular disease that we're looking to target Really in a very holistic way. And it's also, when you think through to who's consuming this data at the end of the day, thinking of what outcomes would be most likely to maybe promote a better marketing strategy or to show that the product performs better than other products that are currently on the market.
Speaker 2:So that is at the macro level, and then at the more specific, I would say the main inputs would be things like what is my expected sample size for the study? What is the amount, what is the duration of time I have to wait before I can have an interim analysis? When is it? When is the best opportunity for me to do an interim analysis and see some of those interim results to inform some other decision during the study? So it really runs the gamut and with all of the new methodologies that are popping up all over the place, over time biostatistics has really become this very rich and fertile ground for adaptations in those studies. So anything that you can think of in that realm is really what we're looking to incorporate into that model.
Speaker 1:Well, there are a lot of different factors that can be incorporated in these models, then, and a very key to both, I imagine, the clinical trials themselves, but also, as you mentioned, how do we position this product in the market?
Speaker 2:Absolutely.
Speaker 2:Yeah, I think that is key and that is something that is sometimes missing and we see that with you know large and small organizations where there are some natural silos, I would say I think that's pretty well known.
Speaker 2:It's not my own critique of the industry, but really when you think of the R&D groups within a pharmaceutical company and maybe the regulatory affairs, and then separately from that, is the marketing arm and the market access arm and reimbursement, health technology assessments and so on, and so oftentimes when a trial is designed in a particular way, the constraints are really within the biostatistical realm, perhaps with medical affairs, with your clinicians or even with the people who forecast your enrollment for the study. But not many times do you really get that crossover with the marketing side to ensure that the level of the data that you're generating is sufficient to make some of those decisions. And so oftentimes when you get to that marketing stage, that market access stage, additional information has to be collected, either through real world evidence or maybe some follow on studies to ensure a proper market access positioning for that product.
Speaker 1:And and Boris, is it new that biostatisticians are involved in these later stages, or have they always been involved?
Speaker 2:So to my knowledge, there's been very little involvement in between the two kind of main silos, if you will. And what part of the vision of products like Solara, which is one of our software solutions here at Seychelles? One of the visions that came along with it is this idea of being able to bring more people, giving more people a seat at the table during trial design, and so we're bringing in the voice of the clinician, the voice of those clinical operations teams, as well as market access and those other considerations, and bringing them all to the same table and having those conversations early on, plus that ability to then simulate at a large scale all of those different inputs, kind of where we hope ensures not only a more robust design, a more kind of optimized design for execution purposes, but then also for that data generation and the use of the data later on.
Speaker 1:And you mentioned a couple of times this at scale part. How big of a scale are we talking about and why is that important?
Speaker 2:Thank you for that. So scale can mean different things to your point. So one way we can think about that scale is the variations in the different inputs into my study. It is also the scale in terms of the breadth of the uncertainties that I'm injecting into that model around my treatment effect, my even my control effect or my enrollment. So those two things add to that scale and that within software like Solare we call these models right, a particular trial design against a particular execution scenario, all those things that we can affect versus all of those things that are outside of our control.
Speaker 2:And then the other way in which this is scalable is the amount of simulation that we run for each one of these models. And so if you think of kind of your general statistics, the more times you repeat that simulation, this calculation of your study, the more confidence you can have in the kind of outcome, of the likelihood of the outcome of your study. And so within a software like East, certainly software such as Solare, you have the ability to repeat the study a thousand times, 10,000 times, 100,000 times to reach a more and more fine statistical analysis of that likelihood of the outcome. Within Solare what is unique is that you're able to take all of those, the three dimensions of that scale as I described it, and put them all together at the same time, and oftentimes I end up with kind of what I call design areas, if you will, of that are the result of millions, tens of millions, hundreds of millions of simulated trials. So those are the outputs from software like that.
Speaker 1:I would be curious to kind of dive into. If we run simulations multiple times, that must give us some sort of range of potential decisions that we can make and how we can design our trials. Many of the executives that we have in pharmaceutical companies have been executives in the pharmaceutical industry for a very long time, and maybe you're not the right person to ask this, but are they ready to make decisions that are more based on potentialities rather than certainties or best practice?
Speaker 2:I think everybody is interested in certainties, but we know in this industry that uncertainty is kind of part of the routine.
Speaker 2:If you will, I would say that most of the time the results of these simulations are expressed as averages, and, again, the more repetitions you have, the closer you are to a likelihood that this outcome is correct. And so I think many executives, many people sitting on governance committees making those decisions about which trials would move forward and which designs to select, I think they are very comfortable with this idea of an average based on simulation. And I think they're even more confident when you're considering a range of likely outcomes and not a single outcome. And so perhaps in the past, when a particular trial was being designed, it was being designed with one treatment effect in mind, and that was, you know, I'm expecting such and such treatment effects. So I need these many patients to reach this power to power my study, you know, at a particular level. And so nowadays we know that there's a lot more uncertainty around that eventual treatment effect, and being able to use the simulation power to get closer to that likelihood, I think, is something that in general people are very comfortable with.
Speaker 1:And what about our regulatory authority friends? Are they interested in this way of modeling trials? What are they saying?
Speaker 2:Yeah, absolutely. And again, monte Carlo simulation has been around for several decades. East Cytel's flagship software has been around for over 30 years and is used by regulators to ensure that the trials that are being submitted to them are functional or optimal to a certain degree, or that they make sense. So I think regulators are certainly comfortable with the idea of simulation. Whether or not there's a good understanding of that combination of simulating at scale and simulating not just a single trial but looking at a wider design space, that's, I think, the area that most of our clients struggle with, and to some extent perhaps the regulator as well, although I'm not I'm not certain about that.
Speaker 1:I kind of want to dive into Solara that you were talking about. Can you tell us more about? I would be interested in the history of the solution. What did it come about to do? What does it do now? How has it evolved? How has it been used?
Speaker 2:Absolutely so. I would say again, cytel has been in this business of clinical trial design, adaptive design and methodology for over 30 years and early on we developed the software called East, which was modularized. It grew over time and East is very widely accepted across the industry. And then in the past five or six years or so I think there was a growing realization that simulating at a larger scale than what East can allow us really opens many different doors and we can spend a little bit more time about why I think this is such a great idea. But East used to be a desktop application which means you were using your own computer's power or your company's server power to run those simulations.
Speaker 2:And I think from some of my colleagues I hear that finding that computational power could be very difficult sometimes in a larger organization, and Monte Carlo simulation does require quite a bit of computational power. So the first thing that Solara provides for us is that computational power in the cloud. In the cloud is maybe a nice way of saying somebody else's computer, but essentially it is connected to, I believe at this point, up to 200,000 cores of computers in the cloud, which means that if I'm designing a trial with, when I'm requiring 10 million simulation runs, solara will fire up as many of those courses are necessary to run that design quickly. So whereas in the past that might have taken us a few days, maybe overnight, to receive some results from a simulation run like that, solara can handle that within 15 to 30 minutes maybe at most. Usually the simulation runs are between 10 and 20 minutes, I would say, depending on on the scale.
Speaker 2:So Solara essentially took the idea of East, which is very advanced adaptive methodology, placed it in the cloud, using that cloud computing power to run those simulations very quickly, and then from there we also expanded into newer and more complex methodologies that are not necessarily currently available in East. So we're providing a wider kind of variation in methodologies, that computing power. And the last thing that I would say is a little bit different is the ability to visualize and communicate results of those to that wider audience. So those people outside of your biostatistics team who may be stakeholders, who may have interests, we have different ways to visualize the data, discuss trade off, discuss what optimal means right. There's different ways to define what optimal is. So all of those things are available within the software and I think those are the three kind of main areas.
Speaker 1:So that's very interesting. I'm also interested to learn more about adaptive clinical trial design in general, because the way that I've been following it so far is that we are getting more types of trials, we're trying more things. Maybe you could take us through. Well, how are we seeing a clinical trial design itself evolving?
Speaker 2:So over the past few decades I think we started with these very kind of plain designs, right, those two arm designs with comparative designs, start to finish. We're not looking at any data in the interim, we're just waiting for those final results. There's a big reveal and we were either successful or we failed. Anything that comes beyond that, in my mind, is adaptive. So introducing an interim analysis to your study, introducing the ability to either enrich your, your, your sample size to a particular subgroup of patients, or just increasing the sample size for the entire population of your study, is another kind of adaptation, also newer ways, I would say, of dealing with multiplicity in your study. So whether it is multiple arms or multiple endpoints which are becoming more and more popular, and multiple endpoints really speaks more again to that piece of how do we make this marketable right? If I can show statistical significance on multiple endpoints, not just say in an oncology trial, not just overall survival or progression free survival, if I can also show statistical significance on quality of life outcomes for the patient, that sometimes gives you that edge in getting that market approval, that reimbursement approval at the end.
Speaker 2:So those adaptations go beyond just the what happens between two arms in a study. So it's dealing with multiplicity and also, more recently, looking at things like basket trials, looking at platform trials. We have several kind of experts within our team who work on platform trials and designs and those are very intricate, very complex. In the future of Solara we are looking to incorporate those types of designs as well, but today those are not part of the software. I just wanted to make that. But yeah, adaptation really runs the gamut. I would say.
Speaker 1:And when we talk about adaptive trials, does that mean we can adapt them as we go, or meaning that we adapt them before we start them?
Speaker 2:Good question. In general, you have to have your study approved by the regulator before you start the trial, and so any adaptation that you're proposing has to be built into your statistical protocols before you hit the ground running. So you may say I want to have an interim analysis, and then you specify in that interim analysis I will stop for early efficacy, but it's this particular boundary that I'm going to declare success, or I'm going to stop the trial for futility. But you have to declare in advance what that boundary would be for that futility decision, if you will. So you can pre-specify adaptations. But if the study is already ongoing, it is much more difficult to make those changes and you often have to go back to the regulator to receive approval for any kind of statistical protocol changes.
Speaker 1:That makes sense If we talk about multiple endpoints that we're trying to measure in the same trial. I'm again curious about the involvement of, for example, market access or HOR in the trial design Because, for example, the quality of life scores. That would be something that we would mainly use for regulatory or for reimbursement purposes later on. How does this change the dynamics of who makes decisions about the trial design?
Speaker 2:Yeah, we're seeing very early signs that certain companies and certain therapeutic areas, I say, are more susceptible to this.
Speaker 2:So if I'm thinking of kind of inflammatory diseases, where we're dealing with many different scales to measure either disease progression or response and so on, or quality of life, where you have a situation where there's multiple scales, that's where we're seeing more and more interest with multiple endpoints Because, again, the more you can show statistical significance on many of those endpoints, the likelier you are, to your point, to receive that reimbursement decision and, depending on the country that you're applying in or the agency that you're appealing to, some favor certain outcomes over others. So at that point, having that seat at the table with the biostatistician early on is where it's most important and it's also, I guess, very dependent on the amount of investment that the company, the manufacturer, is willing to make in that clinical trial, because once you start including more than one or two endpoints, you really have to enroll many more patients to your trial and to get to that finer kind of certain smaller endpoints, if you will down the line as you're using your statistical power to make those determinations.
Speaker 1:If we are, is this something that has already kind of been implemented in our industry, or are we still in different stages, depending on the company, for how well we do with trying to model our trials?
Speaker 2:Yeah, I think in general all again Monte Carlo simulation is something that's very accepted across the industry. I think many. There are many tools. There are many ways in which you can simulate studies or design studies.
Speaker 2:Certainly very common around the industry is using our code as a way to simulate and design trials. I would say that software out of the box, such as Solara or East, has the benefit of sort of tested code. You don't have to start coding every time you want to run a trial. You can just take the solution and run with it. In other ways software can be less flexible than coding. If you're looking to adapt in a very specific way, maybe some cutting edge methodology, there's always this cusp between using software out of the box because it's simpler, it's certainly faster, and again using those cloud resources and that ability to change minor little areas. So I do see a wide use of software, especially our software, across the industry. But in terms of thinking about it in a wider scale, in that larger scale that I was mentioning before, I think that's the part that we're still kind of opening people's eyes to understand the benefits really of simulating at that large scale.
Speaker 1:And besides having software either flexible software that you code yourself or standard software what other things would you need to have in place to have a successful modeling department or area?
Speaker 2:Yeah. So I would say, most importantly, it would be the inputs to that model that you're trying to simulate. So we always say the quality of what you put in is what you get out. So having a good that was a very clean way of saying that, wasn't it?
Speaker 1:That was very. I'm very pissed.
Speaker 2:So having a good understanding of what your competitor or the best supportive care on the market is, that you're probably going to run up against, is very important, and having a good understanding of how your product operates in the world, if you will, how your product affects patients, before you go into the trial. The more information you have the better and then, in terms of applying that Monte Carlo simulation, being able to do it thoughtfully and in a sensitive way to make sure that you're designing a trial that lives up to those expectations.
Speaker 1:And how fast does the methodology in this space move and who drives innovation in the methodology?
Speaker 2:That's an interesting question. It's a little bit of a chicken and egg. So I would say that I've worked in different areas of pharmaceutical drug development. I worked in Market Access, heor also in real world evidence and of all of those I would say biostatistics is Maybe runner up, is also real world evidence, but very academic in nature and very collaborative in nature, and that is something that I really love about this part of the industry is that there's always room for a very open and honest conversation about methods, about how to do things better, about how to be more accurate, and so that's an area that I really appreciate, and I think a lot of the methods nowadays come either from within industry so being developed by some of the foremost biostatisticians working in different pharmaceutical companies also from academia and in some instances also from within providers of software such as ourselves.
Speaker 2:So, for example, sample size re-estimation or promising zone. So thinking of promising zone methodology was something that was created by one of the founders of Seytel, cyrus Mehta. He was one of the foremost kind of writers of some of the seminal papers that have led us to promising zone types of design, and so it really comes from all of those areas, and then it's always a question of who's going to apply it first, who's going to try and implement this in a clinical trial and see if the regulator is accepting it. But there too I think that collaborative nature, that academic nature really helps the entire biostatistics community to bring some of these methods to the floor, because oftentimes the FDA or other regulators will be part of that conversation, would be part of seminars and academic kind of symposia where these methods are being discussed. So I see it as a very kind of collaborative effort, I would say.
Speaker 1:That makes sense. Are there any disease areas where there's more reason to try different simulations than others?
Speaker 2:I think you need to simulate it all. In any case, it's not a particular to a therapeutic area, because that same uncertainty and the need for that same likelihood of certain outcomes is prevalent across all therapeutic areas. I would say that the level of rigor that is required for designing a phase 3 study is a much higher threshold than maybe designing a phase 1 study that might not be comparative, or a phase 2 that might employ only a few patients, and so the stakes get higher and higher as you're reaching those different phases of development, and simulation has space in all of them, but I would definitely say in that phase 3 is where you would have the highest cost, maybe, or cost savings, from optimizing in a particular way.
Speaker 1:And Bois, you have seen different kinds of functions. You mentioned real world evidence, market access, hr, biostatistics. I would be curious to learn your perspective about. Well, what are some of the misunderstandings these different areas have about each other and what are some of the friction points between them?
Speaker 2:Interesting. I may start a war with some of my friends. This is not a fair question, sorry. I think I wouldn't necessarily call it friction. I think they work on a continuum. There's a continuum of drug development, design even early on, kind of like even selecting certain compounds to develop over others.
Speaker 2:So it's a very complex system. I think the misunderstandings happen when maybe some data was already generated, a trial was completed, and then it's thrown over to the other side, right to that marketing side, and then there's this realization oh, this is not enough for us, or it's enough for certain countries maybe and not others, or it's not enough of a difference in terms of the treatment effect to be better than what's on the market already. So it changes the way they can market and can think through how to get reimbursed for that drug. So if there was any friction I would say it's that. But again, I think Pharma has adapted to generate additional evidence in addition to that clinical trial. So it's not the only point of evidence that they're using to reach market access. That's not to say that there's not space for the two to interact even more. And again, that's one of the main rationales for designing a software such as Solara.
Speaker 1:Mm. That makes sense Was how did you get into life sciences in the first place? What was your journey?
Speaker 2:An odd journey for sure. I actually my undergraduate degree is in history of medicine. I've always been interested in medicine and history and that was great. But I realized very quickly that I would not be a very successful historian. So I went back to grad school and studied health insurance markets and how our medicine is paid for and this is in the time of Obamacare, so I'll be dating myself now.
Speaker 2:Ten years ago is when I graduated, and so I really thought I would go into some sort of a nonprofit helping match people with health insurance markets in the United States, and that didn't happen. I was hired by a startup that was in the market access health technology assessment space, kind of helping put together all of the analysis that was needed to make fair decisions around health technology assessment. So I kind of was thrown into this world of clinical trials and marketing medicine and bringing it to patients across the world, and I just fell in love with it so, and then slowly kind of migrated my way across the evidence generation sort of cycle, if you will. So I feel very privileged that I've had the opportunity, at least so far, to really get interested and learn on the job A lot of these different aspects of epidemiology, biostatistics and even kind of marketing of medicine.
Speaker 1:But that's a great background to have, like the history of medicine, because understanding where we come from as an industry and where, like some of the ideas we had about treatment before, makes it so much easier to understand. How have we evolved from that? I think that's a super interesting area.
Speaker 2:Thank you. Yes, I agree, and I think one of the most interesting things that you learn when you get into that health technology assessment in market access world is how different countries value medicine differently. Yes, and it's embedded in the way that they choose to reimburse certain medicines over others, and you can really see trends. Not only you know Nordic countries versus the rest, but each country has their own mechanism of evaluating and valuing a particular medicine and I think it's very telling about that country's culture, its people and how they value their health and their medicines. To me, that's very fascinating.
Speaker 1:Yeah, that is very interesting. When we first met I was actually surprised that Cytel has software offerings. I was not aware I was always thinking Cytel of more of a provider, of people who do statistical programming or statistical programming services, and now you've also mentioned that it's actually had software products for quite a while.
Speaker 2:So is there anything else.
Speaker 1:I don't know about Cytel that you want to tell me.
Speaker 2:So I would say you're right about all of those offerings. Cytel is the largest provider of programming, biostatistic services and software in the world. It's just that we're such a niche market that oftentimes people you know we could be the biggest in your own little party right, I'm a legend in my own living room, but it maybe doesn't translate in that way. So it's interesting that you say that In addition to our software offerings, which were actually the start of the company, was in generating these types of Monte Carlo simulation. I think at the time it was using C++ I forget which languages were used for some of the earlier software that we've developed here at Cytel.
Speaker 2:But, as you've mentioned, statistical programming also kind of functional service provider if you will. So helping life sciences augment their biostatistics departments with some of our own employees. Also real-world analytics and real-world evidence, as well as some market access and HOR capabilities over the years. And one other area that I think is very interesting is data monitoring committees. So part of Cytel also provides the professionals who sit on data monitoring committees and perform those analyses in the interim or what have you, and help shepherd that trial through. So we're one of the biggest providers of data management as well, I think.
Speaker 1:But I did not know.
Speaker 2:Yes, so we do a lot of things. It is really and this one little department of software, but yeah, we do many, many different things.
Speaker 1:Well, boz, we are going to start drawing to a close and I'm going to ask you the question that I ask all of our guests on the show, and that is, if we gave you the transformation trials magic wand that has the ability to change one thing in our industry, what would you wish for?
Speaker 2:And I can only pick one right.
Speaker 1:You can only pick one.
Speaker 2:I think, as we've kind of had this conversation and we've we've already discussed what a complex industry it is and how hard it is to bring a drug to market, I think it would be wonderful if everybody who's engaged in this process across the evidence generation kind of cycle, was able to take a step back from their work, because oftentimes we're so busy in that moment and designing that particular trial or doing that particular task, and just take a step back in the deep breath and appreciate the beauty of the work that we do and appreciate the moment, how momentous it is that we're able to bring these drugs to market eventually and help all of those patients. And I think oftentimes we are so bogged down with our daily kind of tasks that we don't take a moment to appreciate how momentous that is. So that's my, my wish. But as soon as that is done, everybody needs to go back to work.
Speaker 1:Absolutely but. But you're right, we are not great at kind of pausing. It's always there's always the next trial, there's always the next deadline, there's always the next drug or molecule that we need to develop. I love that wish. I hope it comes true. Thank you. Hey, if our listeners have follow up questions or likes to learn more about what you do or what you do, at the hotel.
Speaker 2:Where can they reach out? My email address is my name, it's just boasaddler at sitellcom. You can reach me on LinkedIn. You can stop by Dallas. I'll show you around. I live in Dallas, Texas. I'm happy to show people around my town, my adopted hometown. But seriously, LinkedIn or email is is just fine.
Speaker 1:That's. That's awesome. Oh Boas, this was a pleasure. Thank you so much for coming on the show.
Speaker 2:Hey man, Thank you for having me.
Speaker 1:You're listening to transformation in trials. If you have a suggestion for a guest for our show, reach out to Sam Parnell or Ivana Rosendale on LinkedIn. You can find more episodes on Apple Podcasts, Spotify, Google Podcasts or in any other player. Remember to subscribe and get the episodes hot off the editor.