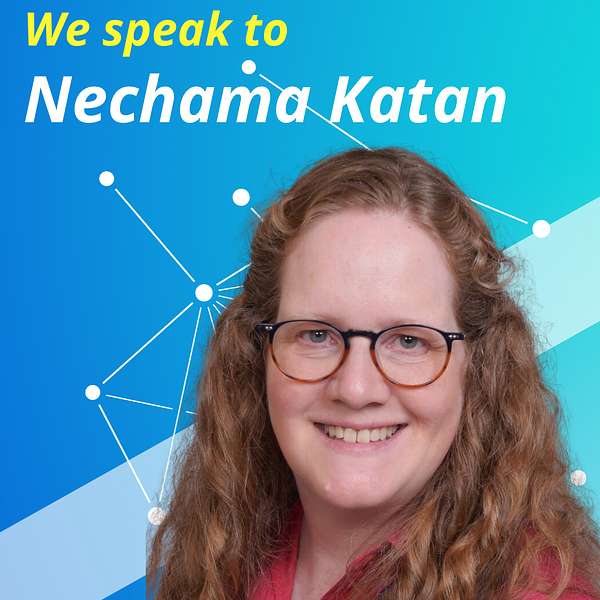
Transformation in Trials
A podcast about the transformations in clinical trial. As life science companies are pressured to deliver novel drugs faster, data, processes, applications, roles and change itself is changing. We speak to people in the industry that experience these transformations up close and make sense of how the pressure can become a catalyst for transformation.
Transformation in Trials
Exploring Generative AI in Life Sciences with Nechama Katan
Join us in a conversation with Nechama Katan, as we investigate the world of generative AI in life sciences, a realm where technology meets business, and where Nechama, an expert in innovative data in the clinical space of life sciences, will guide us.
We're taking a deep dive into the ever-evolving realm of technology in life sciences. We'll take a look at the shift from standalone to integrated tools, and how generative AI is influencing the distribution of labor in life science companies. We'll also explore the potential uses of generative AI in data summarization, and how it could disrupt the traditional role of programmers in clinical trials. Nehamah will share her experiences with chat GPT, Amazon product reviews, Grammarly, and Databricks AI Assistant, shedding light on how generative AI is being implemented in clinical trials and data analysis.
Finally, we will explore technology's significant role in data analysis. Nechama envisions a future where data is readily accessible to everyone seeking answers. She believes generative AI can help us verify random facts and enhance exploratory data analysis. She'll also share insights on how providing the right context to AI can enhance our understanding of the data in front of us. Tune in for this insightful discussion and be sure to reach out to Nechama for any queries or assistance you may need with a complex problem.
Guest:
Nechama Katan
________
Reach out to Sam Parnell and Ivanna Rosendal
Join the conversation on our LinkedIn page
Welcome to Transformation in Trials. This is a podcast exploring all things transformational in clinical trials. My name is Alf Limit on the show, and we will have guests from the whole spectrum of the clinical trials community and we're your hosts, ivana and Sam. Welcome to another episode of Transformation in Trials Today. In the studio with me I have Nehamah Katan, who is a wicked problem wizard and also who does innovative data stuff in the clinical space in life sciences. Welcome back, nehamah.
Speaker 2:Thank you. Thank you, it's great to be here again.
Speaker 1:And today we're going to talk about generative AI in life sciences, some of the challenges, some of the cases that we see and why it's both scary and necessary. And Nehamah first question is where have you encountered generative AI in life sciences so far?
Speaker 2:So we've looked at generative AI in a couple of different cases.
Speaker 2:So the first case is your classic chat GPT, and the challenge with chat GPT is a generative AI tool is that it's open to the public and therefore large companies are very, very hesitant to put their data in. So there's a number of concerns and they're pretty valid about if you put data in and someone query back what are pharma, a company employees researching on chat GPT, for example? That would be the concern. So in that situation, what the larger corporations seem to be doing is taking chat GPT, encapsulating it internally, so you pick up the model, throw it locally and then that model information never goes back outside to the outside world. So that allows you to have a certain amount of control over your environment.
Speaker 2:So that's the first place where we see it. When we think of generative AI, we think of chat GPT, but really we're seeing it in a lot of other embedded ways that you wouldn't notice it otherwise. So let me back out of pharma for a minute. If you open up Amazon now and you ask for a product review, there is a generative AI summary of all the product reviews at the top of it in Amazon. So it's embedded into the tool.
Speaker 1:So yeah, I need to look at that.
Speaker 2:I just noticed it right, so it's in there. It's a generative AI embedded. If I download a tool called Glass on my web browser which I can't do at work, but you can do it at home that will take the transcript of any YouTube video. It will take the transcript of it, throw it into chat GPT and produce from it a summary of that transcript. Okay, so now you've got an opportunity, and I believe that Office 365 Copilot will do this. I do not have access to that yet, but where you could imagine, every meeting at work that you've recorded has a transcript with it with summary meeting notes, the highlights, and you can ask it to summarize the meeting as a blog post, as a memo, as meeting notes, as three bullet points Right, any level of that, right. So we're starting to see these types of integrated tools that allow us to do work.
Speaker 2:Other cases where people have seen generative AI in the past are things like Grammarly. That was actually a generative AI platform. It just wasn't as well known as being generative AI. And the in my case, I'm actually using Databricks has an AI Assistant which allows you to write code. So if you're in a large company, even with the isolated environment, they're very hesitant to allow you to put confidential information into the isolated model. So what most people are using chat GPT for in my space is writing code. Okay, so that's. That's the other example of where we're we're using it, and the challenge there, the things to kind of think about, is where is it integrated and where is it distinct? So if I open up a chat GPT window and say, write the Python code to identify the number of women at a clinical trial site, it's going to say, well, I don't know what the table is. So here's some generic Python code. Okay, and it's not actually bad. Actually, what it's going to do is it's going to write the equivalent of proxy, cool and Python and say call sequel and do it in SQL because Python isn't the language to do it in. But and then it's because I don't know the table.
Speaker 2:So if you go to a modern data tool and again Databricks happens to be the one I'm using where you have a data library, so you click on the table and then you click on the assistant, it's doing two things. One, it tells me what it thinks the table, the purpose of the table is and what each of the columns are likely mean. So it takes all the column names and, based off of its training of data sets, it says we think the site is the study site. Or, if you have, I had a trial where there was like a column where there was like three or four options.
Speaker 2:It said, oh, this was patient status, enroll, discontinued, whatever. So I've read the contents of that column and use the contents of that column with the column editor to make a guess at what that column meant. So you open up the tool and you have on one side your data table, on the other side you have your assistant. Then you can write in plain English Please give me the summary of the number of women per site, or the amount of time, the time difference between two events, and it will find the right variables and it will write the code because it has access to that table, and then it will zap it over to your window and that code will actually run. And so that's the difference between a standalone tool, that just kind of like yeah, I can go through and look at it from a standalone, and an integrated tool. And we've moved since what? March from standalone tools to integrated tools and it's mind boggling.
Speaker 1:Yeah, and also very happening very fast. I'm curious about how this changes the distribution of labor in a life science company, because it seems like we can use more layman's terms, more common language, to actually discover things in data that before would have required more skills in either Python or deep understanding of the data sets themselves. What's your take on this?
Speaker 2:Yeah. So there's a couple things that have changed with it. The first is is that because I can write it in natural language, I don't need to be a programmer anymore. I need to be able to read programming. So in the life sciences we have a lot of people I call them Duel lingo, dual lingo, python programmers or SQL programmers, right? So people who kind of picked it up on the site from an app, right, like they learned a little bit of coding but they're not really fluent, okay. So if you have to go higher a fluent programmer, that person is invested their career and being fluent in programming and not in the content of the subject matter that they actually have.
Speaker 2:So if you take a business user and you put them in front of a tool that requires code, they panic, they panic and shut down they. Just that's what happens, okay. Then if you take them in front of a tool that is a no code tool, they will create, let's say, altrix I'm just using tool names just because they're right. There's this cute little path of my data flow and it's a cute little visual thing and it's drag and drop and it's completely not portable. Because now they're creating something that you can take out of the tool and run it into a data stack and, in fact, if you can write SQL, you hate those because you can't figure out what they've done. Can't figure out what they've done.
Speaker 2:You've seen this? Okay, you've seen this. So I've been democratizing data forever. So with this tool, for the first time, I can take a data set and an expert and have the expert ask the AI assistant how would I write a query to query this data? And I have an exploratory analysis tool in hand, just using Jen AI. So in February I went to a conference and I went to every vendor and said if I have to do gen exploratory analysis, what tool should I use? And they're like we don't know what you're talking about.
Speaker 2:And I said wow so I went and looked for tools and I looked for tools and there's a couple of tools out there. One does graphics and one is data munging, and I couldn't get them to work together. And the big vendors don't have anything and they say, well, go use a BI tool, because data engineering, which is the taking data sets and making sense out of them and merging them and munging them, and data munging data wrangling has always is the last bastion of it. Okay, it's gone. It's gone as a bastion of it, because now, with a tool that has access to the data and this assistant, I can now write that perfect code, but good enough code to start to access my data, and then my good enough proof of concept code can be handed to a programmer to optimize my low, my no code solution could never be handed to a programmer Because, like they're like well, why are you giving me Lego blocks? Just give me plastic beds and I'll make my own Lego blocks, right, and this allows you to then build a jubilee notebook or something else in open source that could then be taken to any tool and be optimized, and so that's. That is completely.
Speaker 2:This tool just got released in the last month is completely transformed how we're going to build our business or teams, everything. It sounds like programmers love it. The program is love it because the programmers have been sitting on their phones texting right, how do I write this? And Python, how do I write?
Speaker 2:this because nobody can remember all the right syntax for all the right whatever's, and so they can use it even better. So it's it's democratizing data engineering. And data engineering is is that getting your data munched, so you have the right data sets put together so that you can then graph it and display it. Graphing and displaying data is something that we've taught school, is children to do. Now, like third graders are doing it in Google sheets, so that's easy.
Speaker 2:But getting that data put together, why I need to merge this data set to that data set and what should I worry about it? With a little bit of training and a little bit of open mindedness, the tool will get the most of the way through the code. So they have to be able to read code Kind of vaguely, like you go to a foreign country and you're like, oh, that looks like shampoo and French, like it's close enough, right. So you have to be able to kind of read code, but you don't need to be able to be a full speaker of it to use a tool like that. And the barrier to reading a language, or to very to understanding a bit some pieces of a language, versus speaking. It is a huge difference, right.
Speaker 1:I'm wondering what this means for the content knowledge or the knowledge of how things relate to each other from a business perspective. Do we need more clarity on that? Now, instead of having specialists who understand you can say the technology part and specialist who understands the business context the business context specialists can now do part way of the technical tasks. What does that do to the purely technical specialists and what does it do to the content specialist?
Speaker 2:So the right measure is to take a technologist and marry and attach them and embed them with a business specialist A curious business specialist, because a curious business specialist at best is going to produce a proof of concept. So before they drew something on a napkin, okay, and that had no tie to the actual data. And then, when the actual data came in, what came out from the napkin and what came out from the data had nothing to do with each other. Okay, so now the business specialist can go from a napkin to oh wait, I've got a little bit of code here, that kind of sort of work, and then you give it to the programmer.
Speaker 2:At the same time that I'm doing this democratization, I'm working with a team of programmers, and programmers who know and really fluent in a language are magical, right, because they they're not afraid of it and they can just build it. So you do need both. You need both, but it's going to connect them because it's there's an overlap. So I went to an event where someone from HP said that the trick with having technology in the business in the same table is that in order to have part of the conversation, you need to understand 30% of each side. So the technologist needs to understand 30% of the business, business needs to understand 30% of the technology and I think that this generative AI helps fill some of that 30%. Maybe it's giving 10 of that 30%, but it makes that barrier easier.
Speaker 1:I like the representation of that. I'm imagining that Venn diagram and how that overlap is growing because there's more bridging between the two domains.
Speaker 2:There's tools that will translate right Gena and I will translate between the domains, and so it's bringing them closer together.
Speaker 1:And that's interesting. I'm wondering, though, because programmers for a while now, back many years ago, when I started in the technology space, people actually wrote their code from scratch. Then we had libraries where you can take big chunks of code and reuse it for different purposes, and now we are having code generated by some of these tools. Will there be a point in time where we don't even need programmers? Can we also generate code for different purposes?
Speaker 2:I think that we can let our programmers do more interesting things than just write code. Right, so we didn't get rid of programmers because we had libraries and we're still using the libraries. If you go to Chet-CpT and you ask it to do something, it pulls up a library. The first thing it does is this is the Python library for you to use. So every point we've said, oh, we've got more technology, we're going to fewer people. No, we have more technology, so we're expecting to use do much more work. Right. So in clinical trials in the past, there was first SDV, sdr, right, and then it's centralized monitoring. So now let's take all this data into a ton more analysis. So now it's going to be all that plus auditory and metadata. We're just growing the amount of data that we're running analysis on and we have kinds of analysis we're expecting to have and the power of the analysis is just growing faster than the technology. So, no, I don't think we're going to get rid of programmers. We're going to get rid of people who just want to program.
Speaker 1:We don't even have typists anymore either People who only type information into a machine.
Speaker 2:We're also going to get rid of business people who don't want to get their fingers dirty in the data, like, oh no, no, don't bother me with the data, but that's gone right. So people both sides are going to come closer together.
Speaker 1:And I think you're right in the development that we are expected, also from a regulatory standpoint, to really have the insights from the data that we generate is no longer enough just to say that, well, this is just the results of the trial. No, it's also about well, how did you arrive at those results? What was the process to getting there?
Speaker 2:Yep, what was the process to get there? So there's another use case for Gen AI. So the first one's the programmers and it's a cheesy one, but it comes with relatively little compliance concern from legal Okay, much less, because you're just doing data engineering generally off standard data systems. Right, everyone has the same five EDC systems. You know there's nothing confidential about your EDC system. So the second use case the Gen AI does a really interesting job of is summarizing information.
Speaker 2:So in a typical clinical trial, you identify your risks and I'm going to go out on a limb and say that most risks live in risk universes or racks systems, risk assessment tools and they can put on a shelf. And I think it was E8R1 that said no, no, no, pull it off the shelf and look at it on occasion, but it's still a risk in a system. Okay. Then you go and you generate a ton of data and all that data becomes issues or signals, right? So the issues are signals, depending on where you fit, or findings.
Speaker 2:If you've raised the issues or signals and now you have on a regular clinical trial, you can have a thousand of those, hundreds of them for sure, hundreds of them, possibly a thousand of them, and any given set of analysis, because you have them coming out of your edit checks, you have them coming out of your monitoring visits. You have them coming out of your central monitoring platform, out of your each of your systems, right, every one of your, your AI machine learning systems. Every system is creating your queries, right, like you've got them right. You just got tons and tons and tons of issues and you have an issue swamp, this quicksand. Nothing ever comes out of the issue swamp.
Speaker 1:I love that image the.
Speaker 2:SCDM paper takes the issues and you're supposed to go back up to continue some improvement to your risk. But in fact you're stuck in this quicksand swamp of all of your issues. And if you go to your study teams and say, okay, give me the summary, what's wrong in the trial? The answer is I have 300 edit checks, data review data checks and 600 risk-based monitoring signals and and right, whatever it is right, some huge number.
Speaker 2:It doesn't matter how many it is, because more than 50 in your human brain is gone. Probably more than 10 in your brain can't keep track of it, so, but each system is producing between tens to hundreds of these sorts of things, and so you've got these all here, and the question is how do you summarize that into one cohesive picture for a site or for a study? And that's where I think Chet-GPT is going to bring the biggest value, once we open it up and figure out how to use it, which is taking the output from all of these issues, aligning them to all of the risks and saying, okay, these risks are still seeing issues that are aligned to this risk, which means by the way we did mitigate that risk, or I have a bunch of issues that I can't align up with any of my risks.
Speaker 2:Let's go review my risks, right? And how can I line up all of my issues so that I can then go find out if they're actually findings? The statistical correlation is never causation, so if I go find a bunch of stuff, can I go understand what happened? So now I need a monitoring visit and site audit something, right? I need some conversation with the trial execution people actually working it to understand what's going on there. So I think there's huge opportunities there in summarizing data and the advantage of using Chet to summarize data.
Speaker 2:Said that hallucinates much less Chet-GPT hallucinates. So if you say, hey, what is a good bio for Avana, it's going to come up with a bio for a typical person in Avana's role, right? So it's completely hallucinated it. If I say, here's everything Avana, here's every YouTube, every video transcript of everything Avana does, tell us what you think about, what are the issues she's concerned with. It's going to come back and say here are the issues she's concerned with, based off right Summary's in all of the transcripts from every video you've ever done, right. And so I think that that's where people talk about Gen AI for drug discovery. I don't see it. I think that, but for issue management and for trying to summarize problems and writing things in clear language so that we can understand them. Yes, that for sure it's going to. It's going to make a big difference. On.
Speaker 1:I think that might be the largest misunderstanding of what this technology can and cannot do, whether it can actually create something out of nothing completely, or whether it's a tool for taking large amounts of something and getting it's clear or answering specific question about that amount of data.
Speaker 2:So even if it's creating something out of nothing, it's creating something out of a huge amount of data. So the question is do you control the inputs to say create out of this, or do you let it just create out of everything?
Speaker 1:I would be curious, because earlier on we talked about that the way that life science companies are working with chat, gpt right now is to create these small universes that are hedge from the rest of the world. Are there any downsides with creating each our own islands with Gen AI, or could we benefit from working across company borders?
Speaker 2:So I think that we should be working across within industry groups, so transcellerate fears, groups like that, because really I don't know that I want to train my best practices from one pharma company. I would much rather train best practices from all the pharma companies, all the life science companies, right? I don't want to do best practice from one, because I know that there's a lot right, you're going to be a much better picture. You're also going to have a bias. So if you take a largely US based company, then most of the drug trial data will be US patients taking European one. You're going to get more European one. Someone in China is going to have China right, and so you're going to have different types of data, different types of issues, different ways of communicating.
Speaker 2:We have global teams now. So I think it's I think it makes way more sense to not have a single sponsor building their own models, but to do the bigger models. So every look. Translate isn't that old. We managed to do translate and we did fuse, so I don't see why we couldn't do a common large language model for clinical trials.
Speaker 1:Another question that comes to mind is since February, since March, a lot has happened in this space. How will we in life sciences tend to move pretty slowly when it comes to adoption of new technology? We're still figuring out how to work beyond paper in some cases. How can we follow along this very rapid development?
Speaker 2:So I think there's two thoughts there. The first is that some companies in the life sciences have tried to skip from having some data to doing AI machine learning Right, so you go straight from one, something that you can't. You really do need to go through each step. You have to step through one to the next, the next. So I think we can play with it in safe and safe ways, to start with, work out some use cases, watch what other industries are doing, and then making sure that in the meantime, we have the right data Accessible for when we can plug it to one to it. So that's the first thing. The second thing is we can push for industry collaboration, because I think if we had industry collaboration going on, then we would be much more.
Speaker 2:Farmer companies are not IT companies, so let's have some industry collaboration around. What kinds of tools we want to do this, use this data with. How do we get industry standards so we can put data around? That all of this requires having access to data, and so and data access takes time Like if you can get your data systems updated to get new data in in less than a year, you're like, oh my God, that's so fast, but hey, you're not going to get it in. So the question is can we work on getting the data access? And and then let's think about not the? The open up chat should be teams. That's fun, but these integrated tools where we're not trying to teach them everything. So the data bricks example is just teaching a data engineering Farmer, can teach it just pharma, or can teach it just clinical trials or just for space monitoring. We can limit the knowledge we're trying to teach it and then it will come up with a much better set of answers.
Speaker 1:That's an interesting thought. That's, this embedded generative AI is probably closer to transforming our industry than the general generative AI. But it also makes me think will the existing vendors, at least the ones who manage to integrate this into the technology, will they just would in fact be a status quo, where everyone kind of adopts this new evolution of technology and but then nothing really changes in our whole landscape of systems.
Speaker 2:Why would it not change?
Speaker 1:Well, if we imagine that our, our EDC system now also has generative AI capabilities and our statistical computer environments also has to be a capabilities, so they remain the same. They just they've just leveled up. You can say they can do more advanced things, but they're still siloed and potentially not integrated. Do we? Do we gain across the board?
Speaker 2:We still need to integrate. But let's talk about this statistical programming, gen AI again, summarizing data is great. Why can't it write a CSR? Right, this project's going on in the industry to have generative AI, take a list of tables and write a clinical submission report. Yes, that's that's again, that's summarizing data. So now that just takes a whole thing and a shrink set. So now the question is what are those people going to do? They should hopefully be thinking about cool other ways to do other types of stuff with the data, or maybe they Data management.
Speaker 2:What does data management really do? Data doesn't get changed. It doesn't change in a clinical trial, and so what does data management do? Data management should do is identify issues at trial sites. Well, that's really study management, and in some companies, study management data management sit together. Right, but if we made data management fast, easy and cheap with the use of these tools, then we could go spend our time having conversations with the study, with the sites, about why site behavior looks different or why do we have protocols that consistently can't be implemented. Or why do we have an?
Speaker 2:E-Pro. That never makes any sense. Or do we have certain types of assessments that sites struggle with? We don't know that because we can't pull that data now, but if we could pull that data we could find all that information. Is there an industry best practice for how to stack assessments? We could do all kinds of really interesting eye level pictures, but really right. So that's to me. It's not that we're going to keep status quo, it's that a embedded tool is going to one, scare people less and two, it's going to be much more relevant to the problem at hand. So it's going to be more focused. Right, amazon's product review we'll go back to that as an example. Just takes the product reviews listed for that product. Doesn't take every possible product review on a can opener. It takes the product reviews for that can opener and it summarizes them. And so that's the power of the embedding. Is that without embedding, without giving the tool context or without giving the program a context, right? So how many? You do technology? I do technology. Without context, your projects fail.
Speaker 1:So it helps us a part of the way with making sense of the data that we have in front of us, but it does not avoid us from having to use our brains in making sure that it actually makes sense in the context that we're trying to use it in.
Speaker 2:It's a way of giving you context to a problem. So if I make sense of the context of my fingertips, I should then be able to like well context and then like syntax. So now I can think about what am. I trying to do so I don't it's. I really don't think it's going to. It's going to transform what we do, but I don't, and it's already transformed my life, but it's not going to destroy the world, it's going to be over. So now there are tools like computers.
Speaker 1:Yeah, or the internet, or search the internet, or what church Search?
Speaker 2:Search, yes, yes, yes, yes. Google search, right, I went to a concert recently and there was a no phone concert, and so my son came up with some statement and we were like, how do we verify it? We have to remember to check the phones after the phone. How do I verify some random fact?
Speaker 1:Well, and how? I think this is a perfect segue to the question that we always ask our guests on the show, and that is if we gave you the transformation trials, magic ones that can transform one thing in our industry, what would you wish for?
Speaker 2:Exploratory data analysis. I want to have data at the fingertips and the people asking the questions so that we can really dig into the data and understand what the answers are, and I think we're like one step closer.
Speaker 1:We are getting so close and that's a wonderful wish, nehama. If our guests have follow up questions to you about our topic or yourself in general, or have a wicked problem that needs solving, and where can they reach out to?
Speaker 2:And I think that's the easiest place or at wickedproblemwisardscom.
Speaker 1:Awesome. Well, thank you so much for coming on the show. Thank you very much. Thank you.