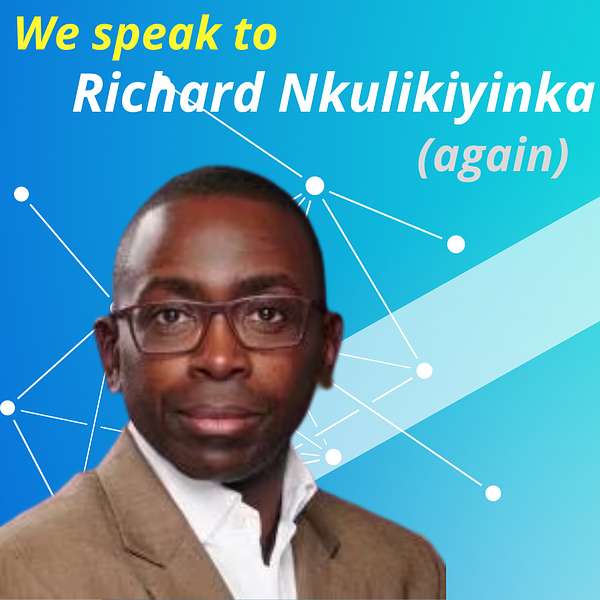
Transformation in Trials
A podcast about the transformations in clinical trial. As life science companies are pressured to deliver novel drugs faster, data, processes, applications, roles and change itself is changing. We speak to people in the industry that experience these transformations up close and make sense of how the pressure can become a catalyst for transformation.
Transformation in Trials
Inventing New Endpoints with Richard Nkulikiyinka
Join us in a fascinating conversation with our special guests, Richard Nkulikiyinka, as we unravel how endpoints in trials are transforming and the significant role they play in determining trial efficiency. We also delve into innovations in oncology endpoints and discuss the challenges and opportunities in cardiovascular trials. Tune in as we explore the use of composite endpoints in heart failure trials and exciting functional endpoints that are potentially leading the way for approval studies.
We took a deep dive into the critical impacts of trial design and data collection on entire trial enterprises, emphasizing the importance of prospective validation. Richard shared intriguing insights about the changing landscape of trial design and its ripple effect on the industry. He gave an in-depth analysis of sodium-glucose co-transporter inhibitors and the revolutionary changes they brought to the standard of care through a series of trials. You wouldn't want to miss their take on the advantages of umbrella and basket trials and the essential role collaboration plays for their success.
In this riveting discussion, Richard also illuminated the transformative power of innovation and collaboration in clinical trials. We delved into the thrilling potential of cross-company collaboration in investigating the same patient population and the possible role of impartial brokers. Richard also sheds light on how AI and technological advancements could enhance clinical trial outcomes. Listen as they dissect the potential of AI in imaging, its role in streamlining assessment processes, offering real-time feedback, and reducing the burden on specialists. As we wrapped up, we examined how machine learning could make clinical trials more cost-effective by automating the adjudication of events in cardiovascular trials. This enlightening conversation is a treasure trove of insights into the dynamic world of clinical trials.
________
Reach out to Ivanna Rosendal
Join the conversation on our LinkedIn page
You're listening to Transformation in Trials. Welcome to Transformation in Trials. This is a podcast exploring all things transformational in clinical trials. Everything is off limits on the show and we will have guests from the whole spectrum of the clinical trials community and we're your hosts, Ivana and Sam. Welcome to another episode of Transformation in Trials. Today, in the studio with me, we have Richard and Kuli Kienka.
Speaker 2:Welcome, richard. Hi Ivana, it's so nice to be back.
Speaker 1:And you are indeed back for a second episode in Transformation. In Trials, sometimes you just have an episode that you record and then you run out of time before you run out of topics, so I'm really happy that you were willing to return and speak to us again.
Speaker 2:Pleasure.
Speaker 1:Now, the first thing that I would like to start us off on is endpoints, because the way that we collect endpoints, the way that we design endpoints, is changing in pharma. Could you help us set the stage for what is happening in this space right now?
Speaker 2:Yeah, I think this is a very exciting and promising topic, but a very challenging one as well, because endpoints really determine ultimately how we design trials and how many patients we need for the trials, how long the trials are, and all of that boils down to basically how much effort needs to go into a trial, how much cost and how much time. So getting endpoints right is incredibly important. That's on the one side. That's basically the as far as the theory goes. Now, in practice, endpoints need to be very robust and I think we chatted about this last time that I was here. Obviously, health authorities, scientists, everyone involved in the clinical trial space has a rightfully so a big interest to make sure that, if we are measuring a treatment effect of some sort with a new treatment, that the effect, that we're going to see all the results are going to be really robust. So everyone wants an endpoint to be reliable, to be reproducible, to be really well established, etc. And that makes it, of course, very you know, somewhat challenging to innovate in endpoints, because innovative or new things by definition, are not going to have a lot of experience behind them, so there's going to be a lot less confidence in how they work. So, that being said, I think in the clinical trial space, colleagues in oncology have done an excellent job over the years and decades at innovating in endpoints because they've been very keen to find, you know, as they started going towards precision medicine, going from calling it cancer to calling it very different types of tumors, to calling even a type of tumor very, in subdividing it in very different types of classes based on the pathophysiology, in particular on the genetics. They also understood that it was very important to have bespoke endpoints for each one of those and there's been a lot of innovation in endpoints there over the years that I think we can learn from in the other areas.
Speaker 2:Now, as you know, coming from a cardiovascular space and that's where really my heart is and that's where I spent the past decade of my work in pharmaceuticals roughly working on, and that is one area where we do excellent trials but we have struggled to really innovate with endpoints, again because of the reasons I mentioned. There are some really good endpoints, mace in particular. So major adverse cardiovascular events is what we tend to look at, and that includes things like myocardial infarctions, strokes, cardiovascular deaths, and there are very well-established definitions for those, on the one hand, that have been really used in multiple trials. So there's a lot of confidence in what you're looking at when you do a trial with MACE and, secondly, the way we analyze these endpoints. Event-driven trials are gold standard again an excellent gold standard and the detractors of it call it calling bodies, so accounting bodies, sorry. Now you do a trial, basically, and you sit back and you treat patients and then you sit back and you see what events happen and you count them and you see what is the time that it takes for a patient to get to that event and you have your result Again very well-established, excellent methodologies behind it. But with time we're realizing that number one we're missing some of the clinical aspects that are very important and sometimes even more important to patients than those events. Give you an example In heart failure I'm pretty sure you know this we do trials that use a general use for approval.
Speaker 2:We use a composite endpoint made of time to first heart failure, hospitalization or cardiovascular death, and that is a very well-established endpoint. But when you and this is what clinicians and health authorities really care about as well they tell you if I'm going to put a patient on a treatment, on a new treatment, I want to know that it will prevent them coming back to hospital repeatedly and that it will prevent the premature cardiovascular death. When you talk to patients, though, quite often what we hear is that, yes, I take a pill my doctor tells me will prevent these things happening, but I don't feel any different, I'm still short of breath, I still can't sleep, I still can't play with my grandchildren, so what is the point? And so, when we think about endpoints, I think it's also important to think about these kind of aspects that are basically putting the quality back into the life for patients, not just adding life, adding years to the life of patients. So, if you think about that, there's a few recent developments with endpoints that are looking at functional endpoints. There's, for example, the KCCQ, the Cancer City Cardiopathy Questionnaire. That is a scale that basically looking at quality of life, and it's been used for a number of years already, but it's only in recent years that we have started with seeing it being used as a main point in its own right, even for potential for approval studies, and that's a very important development, I think. And so that's one aspect looking at things, additional things than just the traditional so-called hard endpoints.
Speaker 2:The second thing, and this is where I think a lot can happen, for innovation in trials is also how we analyze those, the data we collect. The classical methodology is time to first events that I mentioned very well established. I need to emphasize that. But because this is not about detracting the existing methodologies, but they are not the most efficient statistically speaking. And what do I mean by that? You need for the same number of patients that you put through a trial for a certain time point.
Speaker 2:There are emerging methodologies that would allow you to see if there is a real treatment effect much earlier and with fewer patients than the current methodologies.
Speaker 2:And one that I'm really particularly excited about is the so-called win ratio or win odds methodology, which is basically where the group comparison is not just occurring at the level of the entire group, necessarily, but actually you're comparing each patient in each treatment arm to the other patient in each treatment arm and deciding which patient did better on treatment, and if the patient on the treatment and the data, that's a win for the treatment arm.
Speaker 2:If they did equally, then that's a tie and if they did worse, then that's a lose. And that allows you to derive a number of statistics that are much more efficient. To give you an example, we're looking at this now in the chronic kidney disease space and we have emerging evidence we're still working on this and this will be published soon that, looking at past trials that we did in recent years in this space, you could potentially have got to the result you wanted with probably one third to half the patients that we actually needed and with probably a little bit less time follow up time as well. So that would be very exciting because I would, if we managed to really demonstrate that and establish that and get health authorities on board and get these new methodologies established, I think we would be doing a huge favor to everyone in the ecosystem. So let me stop there. I think those are some of the things that are getting me very excited about innovation on end-point trials.
Speaker 1:And what would it take for an emerging endpoint to become a robust endpoint?
Speaker 2:Well, you know a number of things, I guess, and you probably have some ideas if you're asking me this, but I think the two most important things first of all, that it has to be shown, using existing data sets, that this is an endpoint that really replicates what you expect. If you have seen a treatment effect with an existing treatment, can you analyze a data using the new methodology and see that you're still seeing the same result, that you're still seeing a treatment effect there? And if you have not been able to see a treatment effect, does it replicate with a new methodology, for example? Now, of course, you could go into the detail and say you know, maybe it was because you didn't see a treatment effect, because you used the wrong methodology in the past, and now, if you have a new one, maybe you will see a treatment effect. I'd say careful.
Speaker 2:I think the retrospective validation of such a methodology is important. The second thing is then an engagement with the health authorities to get at least a preliminary buying that they say okay, with our neutral arbitration eyes, we look at this method and we think the results that it would deliver would be informative. Those things have to be in place and then for it to really become established, someone has to have the courage to do it prospectively in a trial so that everyone can watch and say this is no longer retrospective but it is actually prospective. So you see it at work and you look at the trial when it's finished, you look at the result and then you see as a result, it's believable from a clinical and statistical point of view. And then from then on it's a matter of basically refinement and at some point the clinical community will get used to it, everyone will get used to it and they will be established.
Speaker 2:And I think essentially that applies whether you're talking about a method for analyzing existing endpoints or whether you're coming up with a new endpoint, like I said earlier, like something looking at quality of life or exercise capacity that has not really been used before. It would be the same approach, I think. Yeah, what do you think? Is there anything that you would also wonder about? Or would that convince you if you were the judge?
Speaker 1:At least maybe I would be curious to unfold the expected data that would create to support an endpoint, because I know that that cascades into exactly what we put on the CRFs and what we measure. Yeah, so if you innovate, if you create a brand new endpoint, I'm guessing that that has lots of implications for your whole trial design. How you collect the data which visits you collect the data at, how you put it in the EDC, how you analyze them statistically it impacts the whole thing.
Speaker 2:Yeah, absolutely so. That's basically where we start this conversation. Indeed, you know, whatever you do with an endpoint ends up affecting everything in the trial, and so it's true that doing the theory in a room with very smart people is easy yeah, I mean, it's not, but that's only the beginning. You then have to be engaged with everyone involved in the entire trial enterprise to make it work, and that's what, basically, what you were describing. That's why the prospective validation is very important, one that everyone will see. Yes, they did manage to actually do all those steps you just described collect the right data, the right data points, be able to analyze them and kind of all converge into a result that we can see, that is robust and it led to a trial that has a good integrity, and then people will start believing. So seeing is believing, I think yeah.
Speaker 1:But that also leads into the next area that I would like to dive into with you, and that's the trial design, because, as we just spoke about, it's also related to endpoint innovation. Then we also have the trial itself. What do you see emerging within trial design, and how is it impacting the industry?
Speaker 2:The problem is, I'm not going to be able to be able to be comprehensive about this. I guess because that's right.
Speaker 2:Yeah, I'm just a humble cardiovascular developer. I have worked in the past, as you know, also in some other areas, in particular in oncology in my early days in the industry, as well as in dermatology and anti-infectives, and each one of those areas of course has a slightly different way of looking at trial designs because that's driven often by the natural history of the disease. That really determines on how you look at your trial design. Take something like anti-infectives you have, generally speaking, oftentimes, particularly when you're talking about antibiotics, you're looking at an acute episode that has a very limited hopefully a limited duration for the patient, but a very critical phase as well where very bad things can happen very quickly clinically, versus something like a dermatology where you have like anti-inflammatory conditions that patients may have from childhood and may have to live with the entire life, with waxing and waning et cetera. So if you go into design trials in those two settings, obviously you're up against two very different kinds of animals and that will determine what you were able to do. But that as an aside, just to say I can't be comprehensive about what is happening in every area, but in cardiovascular and cardiometabolic I think I'm willing to bet that we're going to see more trials that are based on master protocols or similar things, like basket trials or umbrella trials or similar types of approach. Why do I say that?
Speaker 2:Take a wonderful example of innovation, in every sense of the word, that we've seen in the cardiovascular space in the past decade, which was the emergence of the sodium glucose core transporter inhibitors, sglt-2s, and they have truly transformed the standard of care. For what kind of patient? Patients with diabetes, patients with heart failure, patients with chronic kidney disease and patients with at risk of cardiovascular disease. So in general, it's an amazing broad range of patients that these drugs have actually been able to help with one single mechanism of action. How did we get there?
Speaker 2:A sequential and very long way took us there. You know they had to first run files in diabetic patients to show that they can. They have metabolic effects. You know effects on HB1C et cetera. So glycemic control and a little bit of weight loss and perhaps a little bit of improvement on some other metabolic parameters. Then they had to show that these drugs were safe for a long term use, which is where they then realized that actually, not only were they safe, they had beneficial effects on the cardiovascular side. So they had to go back and design trials, prospective trials, looking at cardiovascular event prevention. Those looked good, so they went on to look at renewal event prevention, then to look at heart failure events.
Speaker 2:All the while, whenever you looked at those trials you know there were always subgroups of patients sometimes very significant that had the other condition that was going to be tested then later on in another trial. So in other words, we know that chronically disease heart failure, cardiovascular disease in general, and it's complication, type two diabetes those things are all very close cousins of each other. They like to conglomerate in one patient. So it's incredibly inefficient, if you think about it, to have to do trial after trial after trial with the same drug, the same mechanism, with thousands of patients and several years of follow-up and, of course, huge investments, while patients are still waiting for these drugs to be approved for their particular condition, when you have patients that have all these three things, all of those four things running around.
Speaker 2:So why can't we design trials where we actually look at the treatment effect for several conditions in parallel? This is where the Umbrella trials come in and the basket trials In one type. You basically take one single mechanism and you try to look at its treatment effects on different types of diseases in a patient population. In the other one, you took several drugs that you think might have an effect on a particular condition, but you don't know which one is going to be better, and then, rather than running several trial trials, you put them in a single master protocol and you see which treatment will do best. I'm willing to bet that we will start seeing more of these emerging in the cardiovascular and cadimetabolic space, and this will, of course, require a lot of collaboration. That's my guess.
Speaker 1:So if those are interesting ways to approach a trial and I'm also just reflecting on the term trial in this case, because in my brain a trial is something where you test a specific drug on a specific population and then you see how that works, and that is slow and sequential, where here we have more of a, you have several trials, I guess in my definition of trial happening at the same time. I need to be good at parsing that data out, understanding.
Speaker 2:well, what does it mean when the data that we see yeah, well, you know that's a very astute observation and in fact, let me give you one real life example that I was involved with. That shows why this can work. And we didn't call it an umbrella trial. We didn't call it, you know, we just did it. Basically, we just sat down and thought how can we make this work and the way I would look at it. Just before I start describing it, the best way to describe what we end up doing would be that it was a nested cohort, a nested cohort to study within a randomized clinical trial. So here's a story, similar thing to what I was saying.
Speaker 2:We were looking at a new treatment for chronic kidney disease associated with type 2 diabetes and we had two large international randomized clinical trials running and they were including patients who all have type 2 diabetes. They all had to be on optimal and some center chronic kidney disease, which were very clearly defined, and they all had to be on optimal treatment for both things. Ok, but we knew we had a large range of stages of chronic kidney disease and what we also know is that chronic kidney disease in patients with type 2 diabetes associates with retinal disease in the eye, diabetic retinopathy. So while we were doing the trial, the clinical trial, our colleagues in research were actually looking at whether the same treatment could help patients with their diabetic retinopathy, and they came with results that we thought were pretty promising in animal studies. So, yeah, this drug could actually be really effective also for retinal disease. So the question was OK, do we now start a clinical study in retinal disease? Or how do we get to the answer? Well, we saw in our studies, which went on basically to include 13,000 patients in the clinical trials, there's bound to be a lot of patients with diabetic retinal disease at baseline, and we were collecting this data, but we hadn't planned to do anything particular with it apart from having the information there.
Speaker 2:So we went back and thought what if we tried to find patients who were willing to sign an extra informed consent to provide their data for the original examinations throughout the trial, and then we can analyze those to see if there is a treatment effect also on the eye retrospectively? So this, this, this idea came up while we were trying to try and, and so you can imagine the mechanics how do we do? How would we identify those patients? How do we consent them? How do we then collect those data for the original disease examinations, which were anyway happening because they are mandated by guidelines, how do we design the CRF for this study, etc. So we did all of that and basically ended up with a study within the study.
Speaker 2:So we ended up with a nested trial for written diabetic retinopathy within the larger cardiovascular sorry, chronic kidney disease trials and it was a very smart, I think, way of doing it. We got nice results. They were published. We could see that there was an effect on some, a trend to an effect to fall for the retinal disease. But in all honesty, it was of course also very challenging because of the retrospective nature. But imagine we'd had that information about, you know, from animal data, from the beginning. Then I think you would have been very smart to actually indeed do that from the beginning, but prospectively, and and then avoid some of the challenges that we had trying to basically do it after the fact. But you see, you know that's very real, to show it's not just science fiction, these things could indeed work and be very practicable.
Speaker 1:And what I'm imagining now is that, before running an actual trial, we kind of take a pause and think about well, the data that we're collecting, what else could we use it for, what else could we investigate? Also, just as you mentioned for maybe earlier stages, is there, is there any answers we would like to know, based on this patient population, and then just include them in the trial that you're running?
Speaker 2:anyway, yeah, of course I know I hear your colleagues now telling me well, don't overburden the trial. And they're right. Every data point we collect is going to be, you know, translating to extra monitoring, visit visits and money etc. It's right, that's all correct. But I think it's about finding the right balance, because if the alternative is to say I'll start another trial which will run for five years, cost me a few hundred million, I don't think that's a better way to go.
Speaker 1:But an even more maybe impractical idea that's coming to my mind is well, we as an industry are running many of the same trials where we're looking at many of the similar populations. What if we collaborated on some of those trials and actually investigated things within the same patient population across different pharmaceutical companies, and both got benefit from the data?
Speaker 2:Absolutely. I love that. I really hope that will start happening because all the challenges that we're facing with innovation become more and more difficult to move forward in the pharmaceutical space. They do indeed also have to do with the fact that mechanism for collaboration are not really particularly well incentivized by now. But I would hope that that will change and that we will have honest brokers, if you like, in inverted commerce, like the NIH, like it has done some of those kind of work, but hopefully you know lots of similar honest brokers, neutral parties will emerge that can help multiple companies trying to develop drugs for similar diseases to collaborate and really make the whole enterprise more efficient, definitely.
Speaker 1:But I think you're right, that would require a restructuring of the industry, having that middle layer that is impartial and more like the keeper of the clinical trial, the clinical trial as a container, and then you can input your desired investigation and then benefit from the data. We don't really have that set up, but maybe we could.
Speaker 2:Now there have already been some, some payers, some ways trying to do that and again, oncology here has really the pack. But cooperative groups, you know academic cooperative groups I think could quite could be the right place to go for that kind of thing. But the potential source, things like the IMI, the innovative medicines initiative in the EU, could potentially provide a platform that facilities are kind of things. So there are different ways of thinking about it and I hope the incentives to do that will increase in the foreseeable future.
Speaker 1:Well, now we're talking about innovation within trial design and innovation of the structure of our industry. I'm always also curious about technology innovation and how that may impact the clinical trial space, and especially recently. There has been a lot of hype about AI and now suddenly it is coming and going to change everything. But have we actually seen any applications of AI or machine learning within the clinical trial space and have they had any revolutionary benefits so far?
Speaker 2:Yeah, well, I now I think we're going to have to have another podcast episode. No, but seriously though, I think this is, this is a really exciting area and and oftentimes, when I have these compositions with you know, with friends and family that are not in the clinical trial space but, of course, very well know about health and health problems and having to go and see a doctor, it's a very interesting conversation because they quite often I find myself on the side of you know, of the lonely side of the conversation. Are you really serious? I mean, come on, you're a doctor Are you really serious that you think it would be good that, instead of me having to see a doctor who actually trained and understands a human being, I have to deal with artificial intelligence, with some kind of artificial intelligence system? That's irresponsible, and and I totally understand that and I try to tell people this is something that hasn't quite sunk in and people maybe right, and I think it's really so skeptical that the promise of AI and machine learning is not that it replaces people with smart people, with great training and especially a human touch. No, it's that it enables them to do a much better job. And that's so true and I believe that and I really think in healthcare it's going to be the case all over the place. Here are a couple of examples of what I have seen working in the clinical trial space. This is the initial stages, but I think it will take off and will do wonders for us.
Speaker 2:First example is the topic of imaging in clinical trials, and many clinical trials really depend on doing a series of image. So you do an image, a baseline, to look at how, what is it If you treat the patient and you repeat the imaging to see are they getting better or not? Now these are things that have to be very precise, very reliable and repeatable, and the way we have done that until now is that you hire specialists that do that clinically and have a lot of experience clinically in doing that, and they basically have to assess each image and assign what is the result and we're seeing the described it in the report and then that comes to the CRF and then to the statisticians who will analyze at the end of the trial how that went. So the process number one is error prone, because sometimes the wrong image gets done or poor quality image gets done and it only it's transpires weeks later, and at that point in time you might be too late, because if the scan was supposed to be assessed on at the time when a patient was starting the treatment or at the time when they were four weeks of treatment, and then it goes through the process of evaluation and a week 10, someone says, oh, this is actually a poor quality image, we can't do anything with it. Well, you've missed the opportunity. We will no longer have the four week scans because the error was noticed later. Why? Because you needed a specialist to have the time to look at this image and assess it and basically say that has high quality or low quality. Please tell the site. You have to repeat it a lot. This happens all the time.
Speaker 2:Secondly, it's the time that it takes for the specialist to reassess this. That's a lot of time and that of course adds up in terms of cost and timeline for a clinical trial. So very often at the end of the trials we scrambling to. You know you have your last patient out of the trial and then you realize that you've still got a backlog of, you know, two months' worth of images to actually be assessed. So that adds two months to your trial to actually do the assessments and the reports etc. So it's also a timeline issue. And then the other thing is that we usually have to have several specialists reviewing and then seeing if they agree and if they don't agree then you have to have a third one. So just a very highly specialized and a very cumbersome process Incomes AI.
Speaker 2:In the imaging space it has really been shown that, you know, machine learning systems can be trained to recognize with very, very high accuracy a lot of diagnosis or just do very simple things like measuring dimensions in a fraction of the time of what a trained person would need really a fraction of the time. And I will come to an example in a second, because all of that basically would mean that all you could do, instead of having 20 specialists, you know, supporting your trial and doing all that manually, you could ask the AI to do that assessment. It does it real time. It can immediately show, send a flag to the site that the image quality is not sufficient. You need to repeat this before the patient has already gone home. They can repeat it, you can do it. It's all the quality checks. You can do all the automated reporting in a matter of minutes or seconds without someone having to see their in-dictator report. You can really automate a lot of things while still maintaining diagnosis, and then all you have to do is for someone trained to eyeball the whole thing and just see does this look real? Yes, keep going, keep going, and then you could cut down on the amount of time that you need for these things while not compromising the safety.
Speaker 2:So I got involved in this for echocardiograms, which I was quite skeptical about, because, you know, the echo of the heart is like ultrasound of the heart. The heart is beating all the time. It's a moving image that you have to assess, and so when the first time I heard about this, I thought you know, I can understand that a machine learning system can measure a tumor, the size and position and consistency of a tumor Fine, I can get that. But really echocardiograms I was a little bit skeptical. Well, I was proven wrong. There are some excellent machine learning applications out there that can assess an echocardiogram and really give all the dimensions, give the diagnosis, really assess the function of the heart in the function of the time and we're talking about 30 minutes for someone highly trained, really quick, to do the exam and report it, to basically look at the exam, do all the measurements and report it, versus less than two minutes for AI. Secondly, it's able to.
Speaker 2:Again I'll say and this comes from really painful real life experience that I did a trial where we had to look at echocardiograms at the beginning of the trial for inclusion in the trial. But we were doing, you know, the traditional way that they had to be uploaded into a system and then someone had to basically check the quality later on. But all these things were taking time, both the uploading from the site and the review from the vendor and then the report back to site. So we had loads of quality issues that would be coming up very late. Now we try to simulate what that would mean if we had had the system in place with AI and we could have cut back so much of that, of those quality issues, because this is more than being able to immediately raise a flag. This needs to be repeated. So I think this will definitely make a huge difference.
Speaker 2:And just a quick example in cardiovascular trials, another thing that we spend a lot of time on and a lot of expertise on is adjudication of events. Yeah, where you have, you know, is this a if a site report that someone has had a heart failure hospitalization. How do you know, really, that it was a heart failure hospitalization? Well, the way we do it is that we ask them to send all the documents to us. We give the documents to three experts who look at them and basically say, yes, you know the symptoms, the presentation that the patient had, the symptom and the treatment they received and the results of the bloods, et cetera. Yes, this was a heart failure hospitalization or it wasn't. And if they cannot agree, then you know. It said one has to be called a shot.
Speaker 2:This is again something that you can really automate and the first trials of this show that you could basically correctly adjudicate about maybe 80 to 85, 90% of the cases with a machine learning algorithm, and then the 10 that are a little bit more difficult you can give to this group of people to really have a deeper look, and the other ones are just basically a matter of quick check eyeballing. Does this make sense? Again, something that could cut out time and effort and money out of time. So very excited about that. And it's not replacing these experts, as we hear. They are still in charge of making sure that things work. It's just making their lives much easier and their work much more efficient.
Speaker 1:I wonder if that would impact how we train these experts. Then how do we train them to rely on these tools instead of doing all the work themselves?
Speaker 2:Yeah, well, that's probably for other people to decide, I guess, but that's something I have wanted as well. But yeah, I mean this applies to all areas where AI is applied. Look at all the discussions that are ongoing with charge EPT in schools and universities, etc. They still train people to think critically or to synthesize information. If they have charge EPT, they still need to. I think so, and I think that will not replace the training for these specialists. But you will also have to integrate, indeed, an element of how do they rely on those tools in their work.
Speaker 1:That is still a transformation in progress and that's a good segue to the question that we always ask our guests on the show, and that is, if we were to give you the transformation trials magic wand that can give you one wish that could change our industry, what would you wish for?
Speaker 2:no-transcript. I would love to see what color. Would it be? The magic one? Yeah, yeah, because that might determine the answer. No, you know, if I had a magic one, I think I would waive it to make clinical trials more cost effective, significantly more cost effective, and I know this may sound like obvious, like a truism, but let's think about that for a second, ivana.
Speaker 2:So if you look at where we spend the money in the pharmaceutical industry, in my experience 60 to 70% of the cost of developing a drug is really purely clinical trial costs. That's where the spend happens. And where do we spend that money? On two big chunks. Again, this one in the industry will not be surprised about this. This is obvious. One of the biggest chunks is compensating the sites for the work we have to do identifying patients, putting them through trials, monitoring them, et cetera, et cetera. And the other big chunk is the operations of the trial, the things that we kept also bringing up making sure that we collect the data, ensure the quality, ensure the patient safety with monitoring, visits and all these kinds of things. So the operation costs and trial side costs.
Speaker 2:So if we say we're going to make clinical trials significantly more cost effective, it means that for every dollar or every euro we're spending, we are getting more done. So what does that mean? If I look at it from the side perspective, it can only work. If it works, it means that the site is being able to do more work for the same amount of effort or money, if you like. So that means that we have improved the process, workflows so much that people don't spend whole entire days just managing one patient to a trial, but maybe a couple of hours. So that would allow the sites. That will leave the sites and give them the opportunity to decide either I'm able to include more patients because I can manage more patients in the trial, or I free up time for my physicians and nurses and other trials to actually do clinical care, which is usually often the clash that we have there. So the sites would definitely be on the benefiting side.
Speaker 2:Look at it from the patient perspective. If the sites are able to put more patients in a trial or have basically more leeway, well, that means that the likelihood of a patient who wants to participate in the trial getting into a trial increases because the sites have basically more capacity to take to take on patients. Or, if it's not in a trial. If information for patients are only a trial, the likelihood that we find the treatment appropriate for the disease will increase because we're able to do more trials. So it would be great for our stakeholders.
Speaker 2:Now, looking at it from the industry perspective, that's kind of obvious. I think it would be great if we would be relieved from having to make those agonizing choices that we sometimes have to make, one trial versus the other, based on cost, because money available is limited, or, even more often, one asset versus the other, and we could just be able to make more choices really just based on the merit of a new drug, on the scientific merit and the likelihood of technical success. So I think that would be a great word to work in. I don't know how you see that, but I think it would be a wonderful word to work in.
Speaker 1:I think that could unleash some abundance into the industry if we could make our clinical trials more cost effective.
Speaker 2:Yeah, and I will take basically a confluence of all these things we're talking about. You have to have efficient trials. You have to choose, be able to deploy endpoints that will allow you to be to get to the answer faster and better, while still being robust. You will have to have things that shrink the effort put in the workflow by the individual people in the trial, in the clinical trial, while also reducing the cost so you can deploy the money elsewhere. So it's a lot of things that would have to happen. But you said it's a magic wand. That's what we're using it for. I love it.
Speaker 1:Well, richard, I feel like we could spend another episode talking about lots of interesting things, but if our listeners want to reach out to you and ask you follow-up questions, then what we talked about, where can they find you?
Speaker 2:Easy to find. So I'm on LinkedIn. I'm on LinkedIn there and I think my profile is public, and I always love people reaching out and saying let's have a conversation about innovation trials or any other topic, and my email address is richardn at gmxnet. I guess you'll probably be able to put it in the notes for the podcast. Yeah, and it's indeed really very nice always connecting with people who have the same share, the same excitement about innovation clinical trials, like yourself and Sam.
Speaker 1:Well, thank you, Richard, so much for coming back. It was a pleasure having you on the show.
Speaker 2:Thank you so much, ivana. I wish you a wonderful rest of the summer and, yeah, speak to you soon.